The Potential of Retrieval-Augmented Generation (RAG) in Healthcare
Imagine a doctor faced with a critically ill patient in the emergency room. Every second counts, and making the right decision requires access to a vast amount of information—the patient’s medical history, the latest clinical guidelines, and insights from recent research. What if an AI-powered assistant could retrieve and synthesize all of this information instantly, ensuring the doctor has the tools needed to save a life? This is the promise of Retrieval-Augmented Generation (RAG), a transformative technology poised to revolutionize healthcare.
Large Language Models in Healthcare
Large Language Models (LLMs) like OpenAI’s GPT or Anthropic’s Claude have become an extremely import component in modern AI applications. They at synthesis and generating human-like text, which allows for machine systems to understand complex associations and concepts present in data and transform those into a form that can be acted upon. This capability enables applications ranging from drafting patient notes to generating plain-language explanations of complex medical concepts that weren't possible with earlier systems.
For healthcare, the potential is immense:
- Enhanced Decision-Making: LLMs can provide clinicians with quick summaries of medical literature, synthesize data into actionable insights, and serve as inputs to other forms of decision making software (such as early cancer screening systems).
- Improved Patient Engagement: Chatbots powered by LLMs can answer patients’ questions about their conditions, medication, or treatments, reducing the burden on healthcare staff.
- Operational Efficiency: Automating administrative tasks, such as appointment scheduling or insurance claim processing, allowing providers to focus more on patient care.
The Challenges of Relying Solely on LLMs
While the benefits of LLMs are undeniable, they are not without significant limitations:
- Limited Knowledge: LLMs are bounded by the date of their training data, making them unable to incorporate recent developments in medical research or practice.
- Tendency to Hallucinate: LLMs sometimes generate plausible-sounding but incorrect or entirely fabricated information, posing a risk in high-stakes domains like healthcare.
- Lack of Specialized Knowledge: They lack access to proprietary or internal data, such as patient records or an organization’s unique clinical guidelines.
These shortcomings make it clear that while LLMs are powerful, they need to be augmented to meet the specific demands of healthcare.
Introducing RAG: A Game-Changer for Healthcare AI
Retrieval-Augmented Generation (RAG) addresses the limitations of LLMs by integrating them with external data retrieval systems. The result is an AI system that combines the generative capabilities of LLMs with the precision and reliability of curated data.
What is RAG?
RAG enhances LLMs by:
- Retrieving Relevant Information: A retrieval layer fetches specific, relevant data from external sources like medical databases, patient records, or clinical guidelines.
- Augmenting LLM Input: The retrieved information is combined with the user’s query to provide contextually accurate and up-to-date responses.
- Generating Informed Responses: The augmented input is fed into the LLM, which generates answers grounded in the retrieved data.
This approach mitigates hallucinations, extends the model’s knowledge base, and enables access to specialized or proprietary information, making RAG an ideal solution for healthcare applications.
RAG in Action
The transformative power of RAG in healthcare lies in its ability to provide real-time, context-aware insights by combining external data with AI capabilities. Here are two use cases that demonstrate how RAG can enhance patient care and empower healthcare providers.
Clinical Decision Support
Consider a scenario where a physician is treating a complex case, such as a patient presenting with symptoms indicative of a rare disease. With RAG:
- Retrieval: The system pulls the latest research articles, clinical guidelines, and similar case studies.
- Augmentation: This information is synthesized with the patient’s medical history and diagnostic data.
- Generation: The LLM generates a concise summary and suggests potential diagnoses or treatment options.
Value: The physician can make informed decisions faster, improving patient outcomes and reducing diagnostic errors.
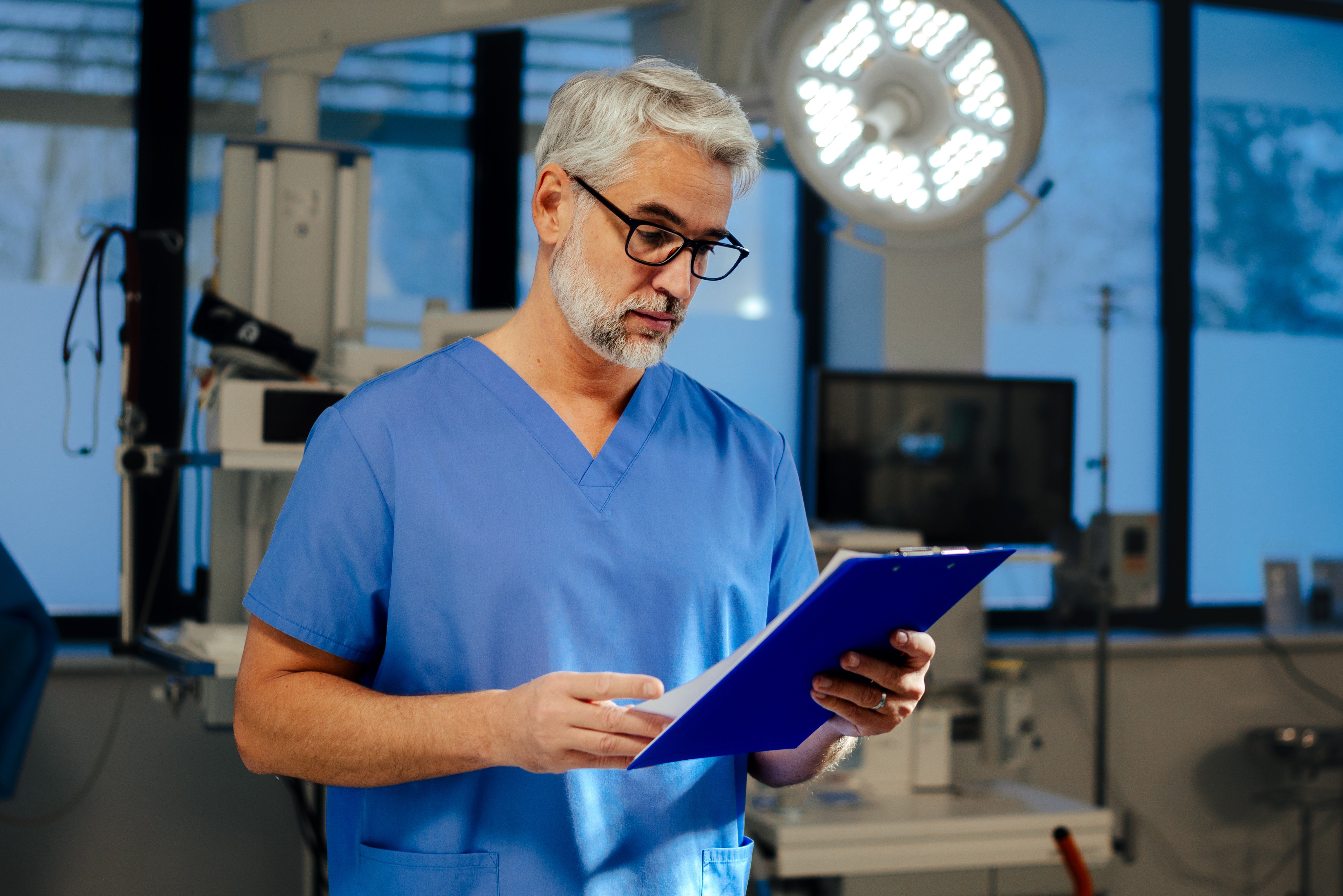
Patient Engagement and Education
Imagine a cancer patient navigating a new diagnosis and treatment plan. They have countless questions about their condition, side effects, and what to expect. With a RAG-powered chatbot:
- Retrieval: The system accesses up-to-date clinical information, patient education materials, and relevant case studies.
- Augmentation: Combines these resources with the patient’s specific medical context.
- Generation: Provides personalized, accurate, and empathetic answers to their questions.
Value: Patients feel more informed and supported, leading to better adherence to treatment plans and improved satisfaction.
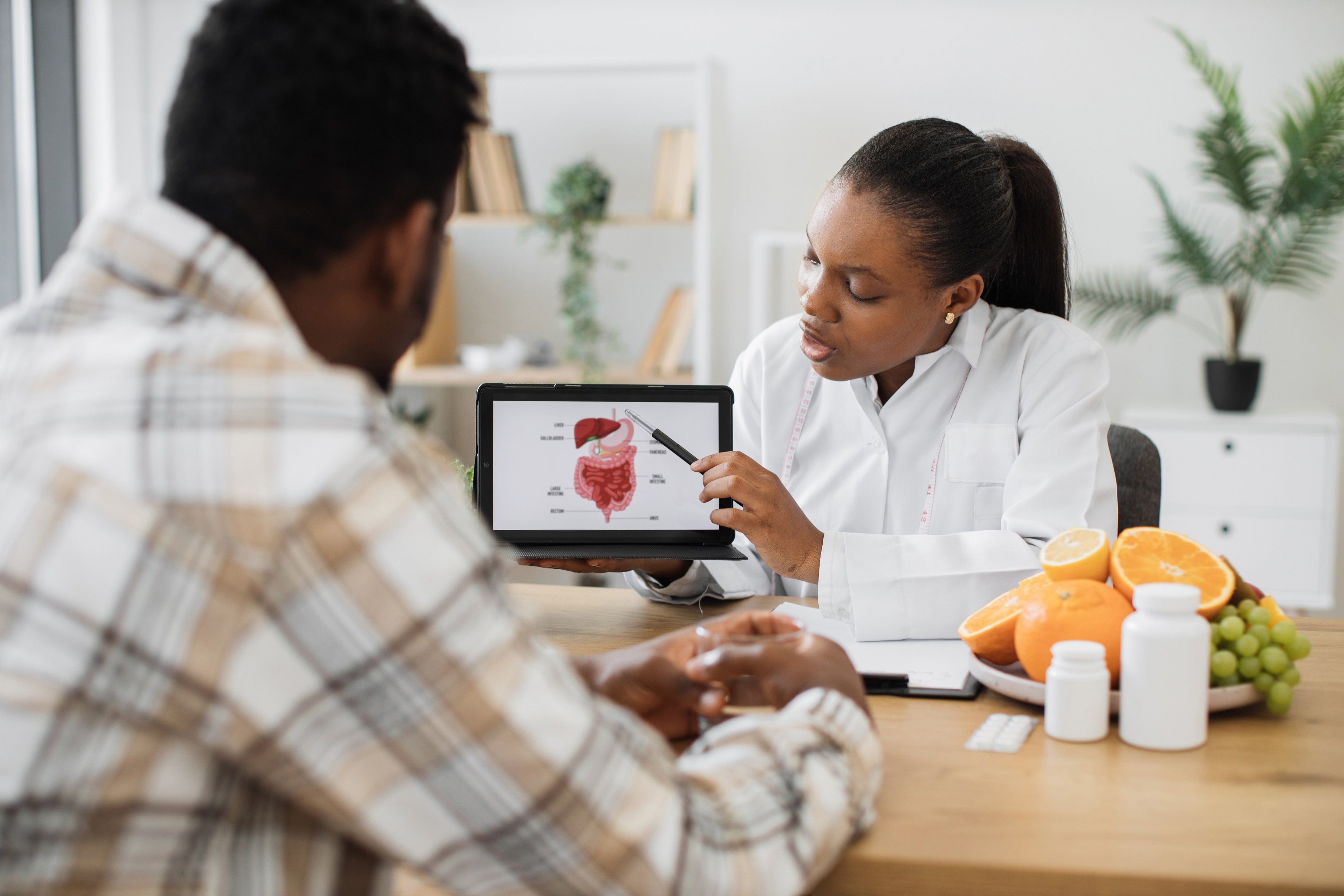
So ... What?
For healthcare organizations, RAG offers more than just technological innovation—it provides a competitive edge. By leveraging RAG, hospitals and clinics can:
- Enhance Patient Outcomes: Equip providers with the tools to make better decisions, faster.
- Reduce Costs: Minimize inefficiencies and streamline operations.
- Build Trust: Deliver accurate, reliable, and personalized patient interactions.
Retrieval-Augmented Generation is not just a technology—it’s a paradigm shift for how AI can be used in healthcare. By combining the strengths of LLMs with the precision of data retrieval, RAG systems provide an unparalleled ability to improve outcomes for patients, streamline processes for providers, and drive value for organizations. The future of healthcare is here, and it’s augmented.
Comments
Loading
No results found