Models of Health
We live in three dimensions, so should healthcare data.
Moving Beyond Flatland
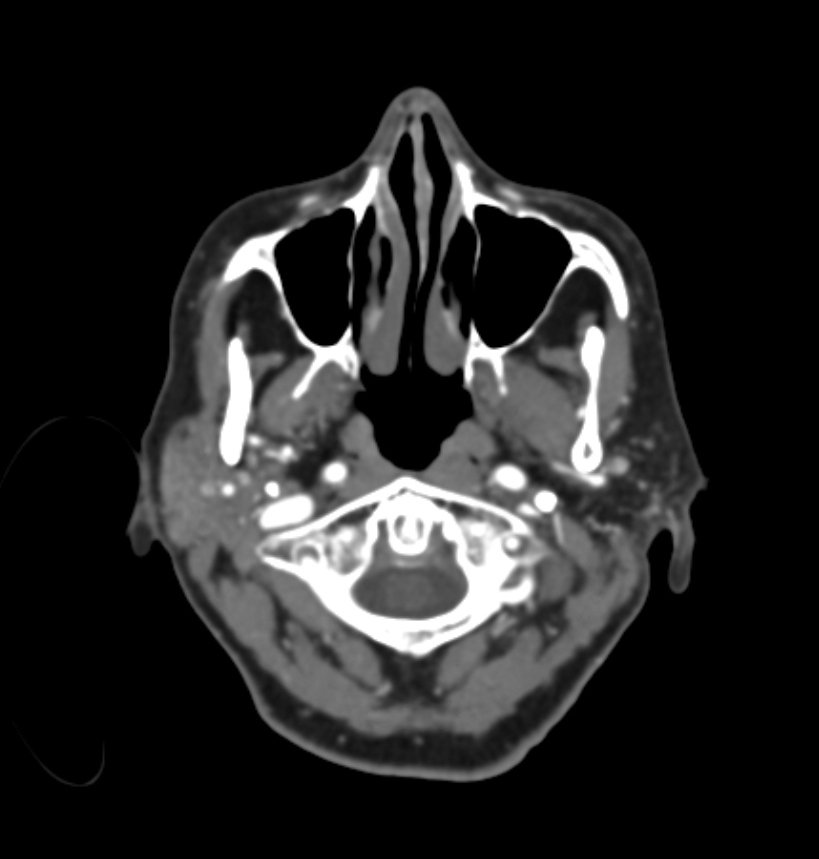
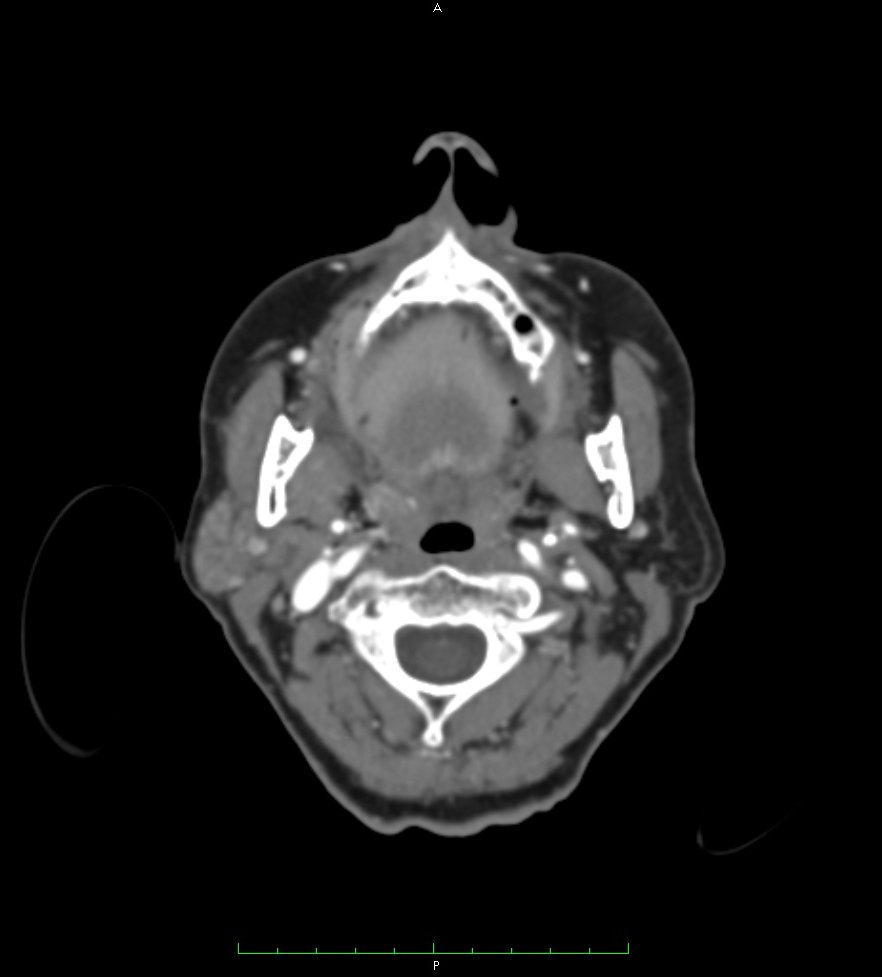
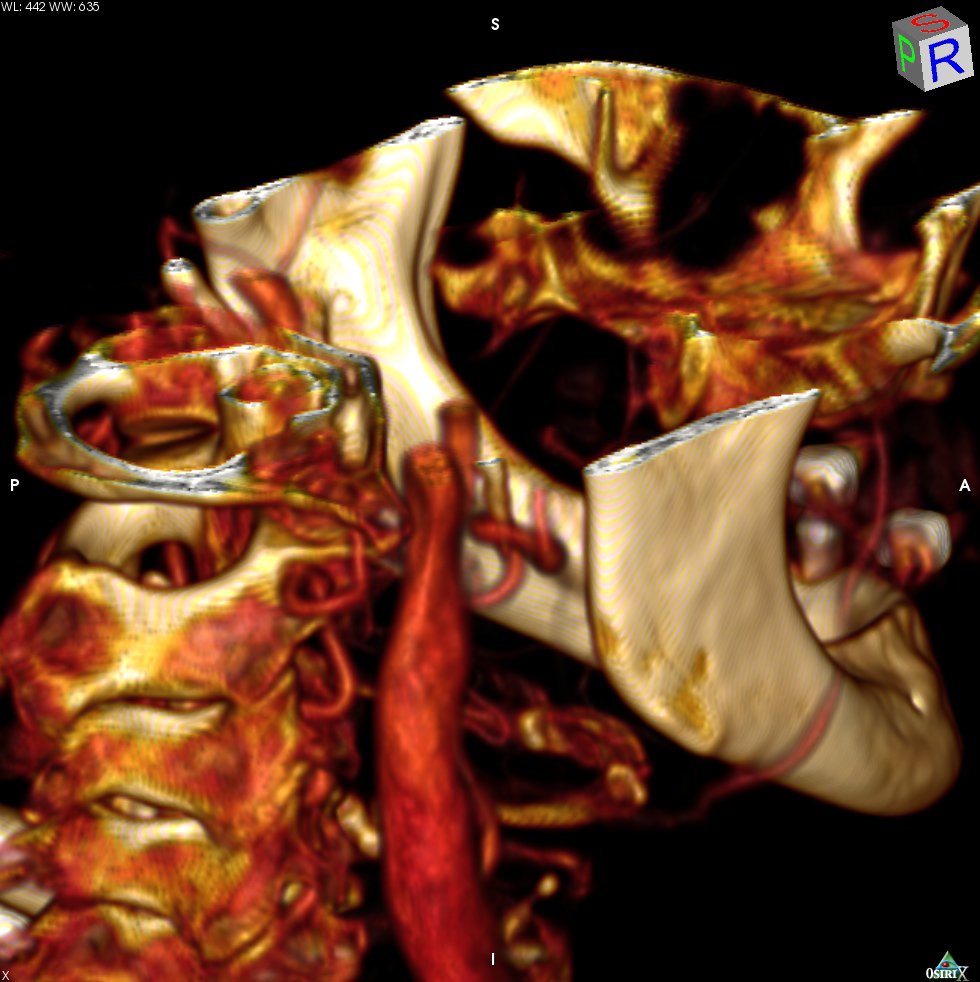
Assessing Atrial Fibrillation Treatment Efficacy
Atrial fibrillation (AF) is the most common arrhythmia in the world and impacts tens of millions of people. Patients suffering from AF live a diminished quality of life and are at risk for other serious conditions such as stroke.
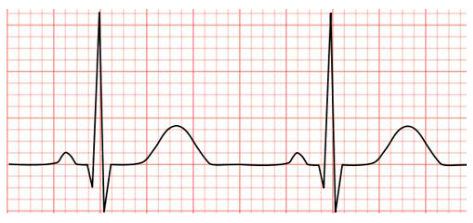
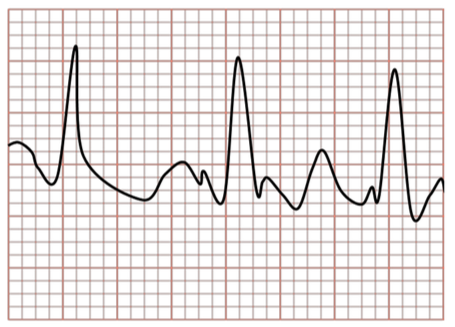
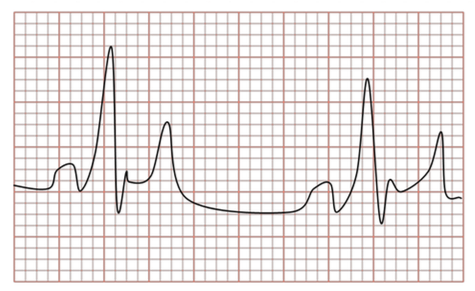
Assess Treatment Early and Effectively
Atrial fibrillation is treated by finding unhealthy tissue and ablating (killing it).
- Ablation treatment is a fine art form. Frequently, tissue is only partially ablated and will continue to contribute to uncoordinated electrical activity.
- Knowing if tissue has been completely treated can be difficult to to assess without an invasive procedure.
- Non-invasive (medical imaging) therapies combine with machine vision can help to quantify how effective a treatment has been and whether additional intervention is necessary.
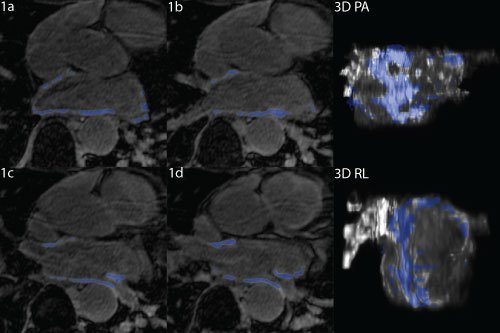
Machine learning allows the building of patient specific models.
It can be difficult to apply new therapies because of how labor intensive it can be to create models outside of the research lab. Machine learning can be leveraged to segment images rapidly and efficiently without losing accuracy.
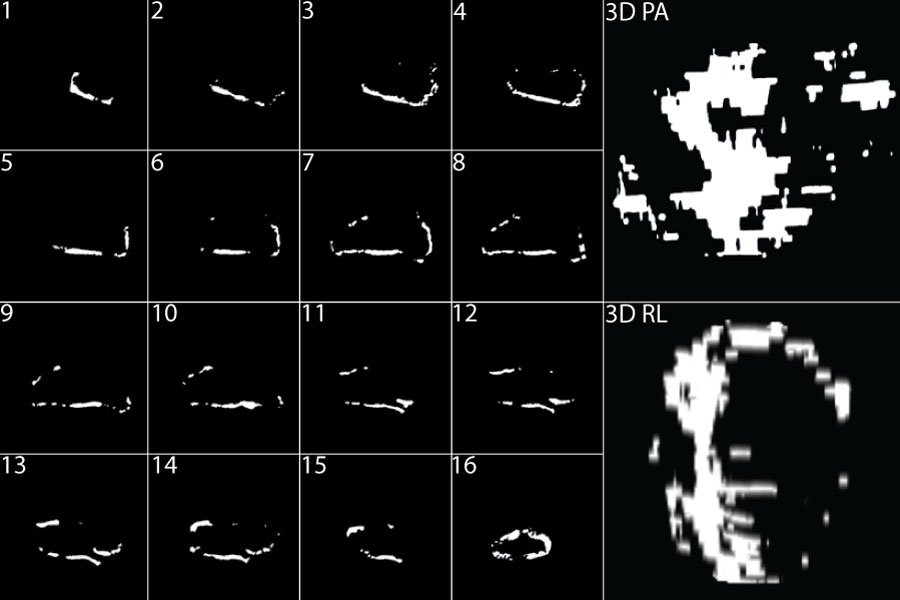
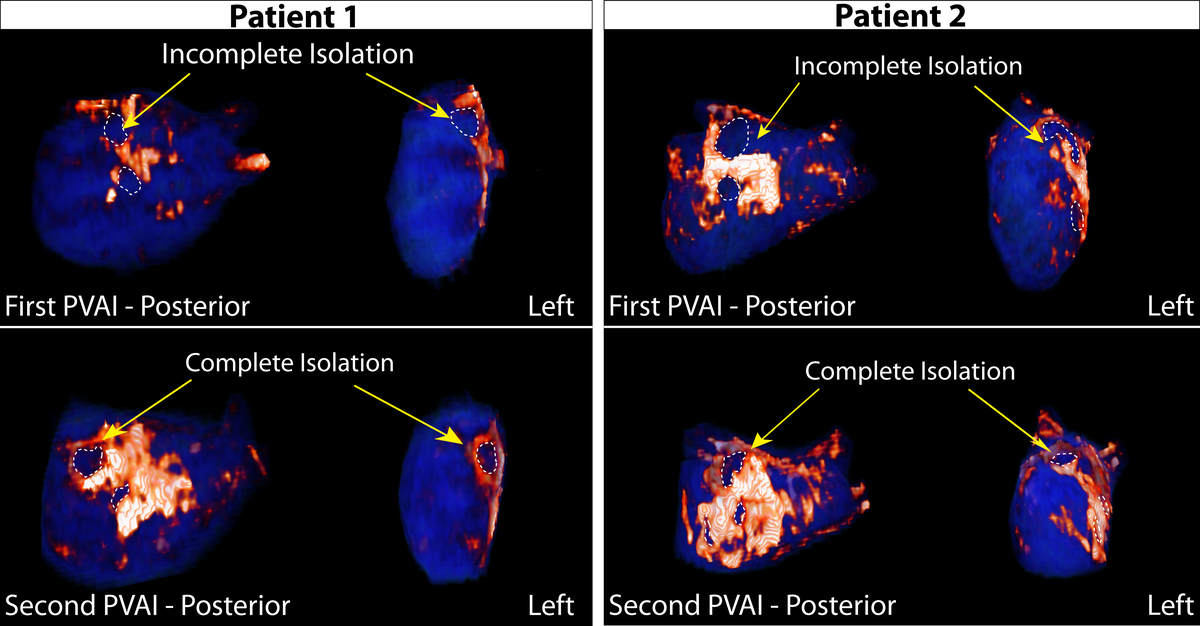
Quantifying the Severity of Atrial Fibrillation
Structural changes in the heart correlate with electrical changes.
These structural changes aren't always reflected in the way the disease manifests; but strongly predict response to therapy. They are really hard to see without an invasive study, though. Medical imaging alongside computer vision, segmentation, and machine learning assessment can give insight to how far along the disease really is. This information then informs treatment options leading to better outcomes.
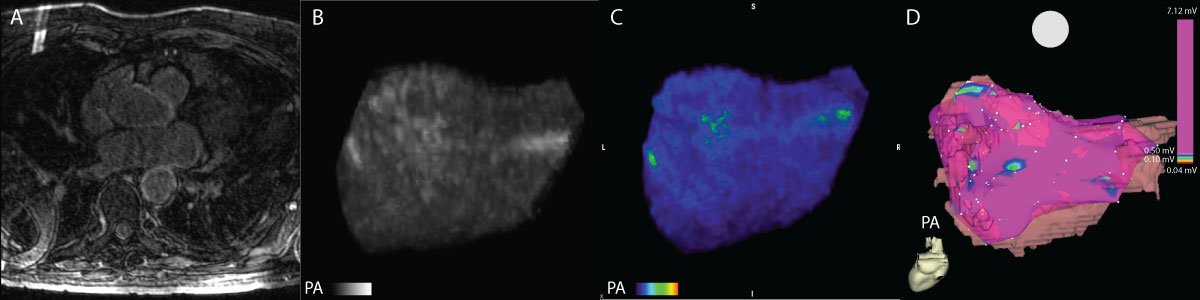
Pictures worth thousands of words.
MRI combined with image processing pipelines provide a powerful tool to inform doctors how to treat arrhythmias. Visualizations can help providers plan intervention and then to communicate findings and care plans to the patient.
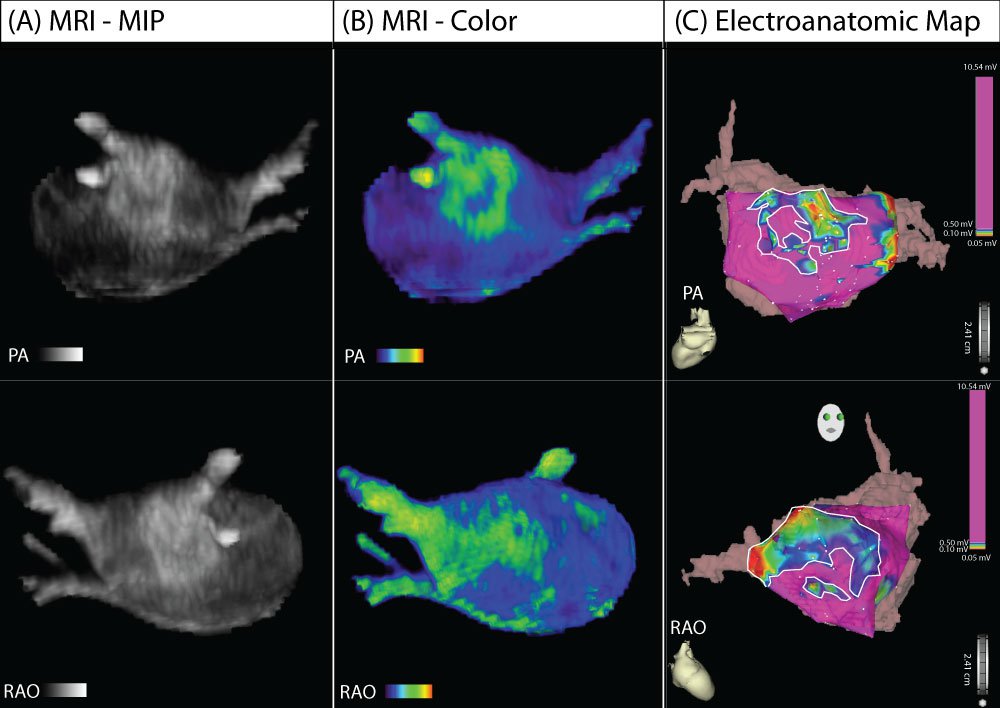
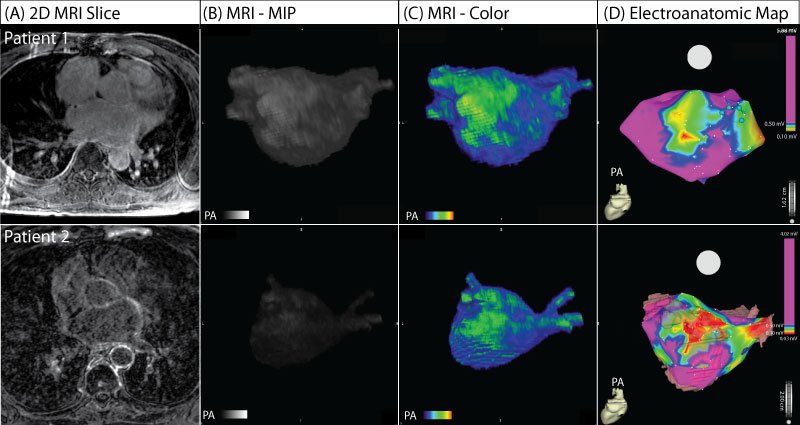
Creating positive feedback loops.
The resulting models provide key insights into how a patient will respond to treatment. Patients with more observed structural change and higher degree of scarring require much more aggressive therapy then those with less change. Over time, as more data is acquired, it is possible to even more effectively target therapy and predict outcome.
Comments
Loading
No results found