Apache Spark for Data Analytics
Apache Spark is the computational engine that powers big data. In this course, you will learn how to use Apache Spark to work data, gain insight using Machine Learning, and analyze streams at scale.
The ability to store, aggregate, and analyze large amounts of data has transformed nearly every industry. Whether finance, medicine, entertainment, government, or technology; the dream is the same: use enormous amounts of data to understand problems, predict outcomes, and take effective action. While many advances make the dream of “big data” possible, one of the most important components of the technical stack is the engine that provides the “distributed computing."
In many organizations, Apache Spark is the computational engine that powers big data. A general purpose unified analytics engine built to transform, aggregate, and analyze large amounts of information; Spark has become the de-facto brain behind large scale data processing, machine learning, and graph analysis. It has seen rapid adoption by companies such as Netflix, Google, eBay, and others to analyze at massive scale processing petabytes of data on clusters of thousands of nodes.
In this course, we will explore how Apache Spark can be used for data processing. We will cover the fundamentals of Spark including the architecture and internals, the core APIs and data structures, and how Spark can be used for machine learning and analyzing streaming data sets. Throughout the course, you will:
- Understand when and where to use Spark.
- Leverage strategies to create data-driven questions that can provide scientific or business value.
- Learn how to use Apache spark to load, summarize, query, and visualize structured and semi-structured data.
- Introduce common machine learning techniques that can be used to solve supervised and unsupervised problems inside of Spark.
- Learn how to analyze streaming data using Spark Streams.
- Gain hands-on experience with techniques for deploying Spark as part of larger software system.
Target Audience.
- Software engineers who are seeking to understand Big Data analytics and extend their skills.
- Data scientists and analysts who need to work with data at moderate to large scale.
- Data and database professions looking to add analytics skills in a big data environment.
Prerequisites.
Participants should have a working knowledge of Python or Scala and should be familiar with core statistical concepts.
Objectives.
- Introduce the components and data structures of Apache Spark and describe how they are used.
- Demonstrate the differences between resilient distributed datasets (RDD), DataFrames, and Datasets.
- Show how Spark be used to ingest data from different types of sources (files, databases, and other storage technologies) and how the data can be transformed, combined, aggregated, and analyzed using SparkSQL.
- Introduce the Spark machine learning library, SparkML, and show how supervised and unsupervised machine learning techniques can be used.
- Show how tools such as Natural Language Processing (NLP) can be used to perform classification or predictions using unstructured data.
- Discuss streaming and how it differs from traditional batch processing. Demonstrate how the Spark Structured Streaming allows for the analysis of datasets that never end.
Course Details
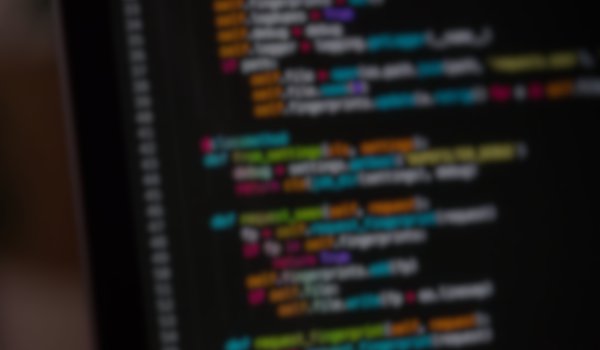
Day 1: Python
Session objectives:
- Review the fundamentals syntax and structure of Python.
- Learn about Python libraries for working with and visualizing data.
Modules
- Mining Data for Value
- Python for Data Analysis
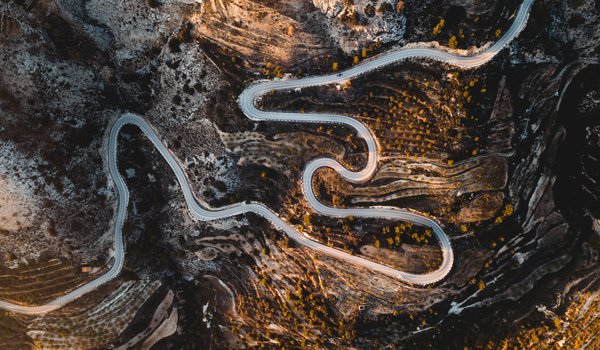
Day 2: Data at Scale
Session objectives:
- Understand the Big Data ecosystem
- Learn the fundamental pieces of Spark -- Spark SQL, MLlib, GraphX -- and when to use them.
Modules
- Spark SQL: Structured Data Fundamentals
- SparkML: Machine Learning in Spark
- Spark Streaming: Analyzing Datasets That Never End
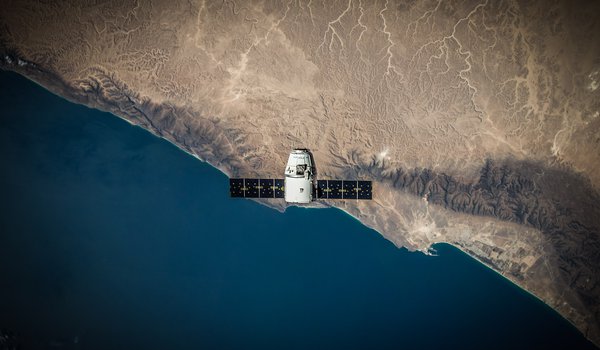
Day 3: Spark in Action
Session objectives:
- Show how Spark can be used to solve data science challenges
- Show how tools such as NLP and deep learning can be used to work with unstructured data
Modules:
- Case Study: Natural Language Processing
- Case Study: Looking for Cancer (Machine Vision)
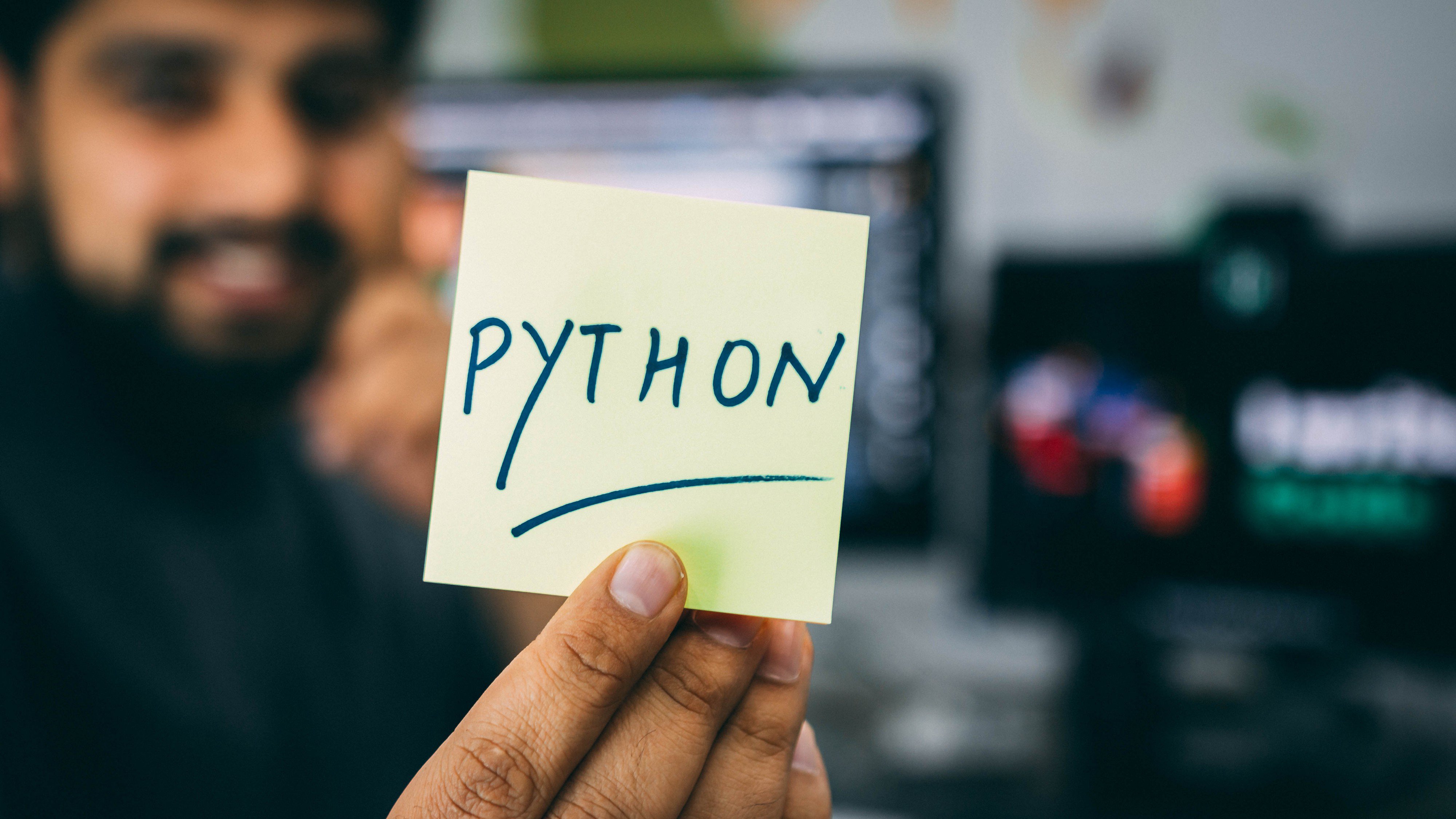
Mining Data for Value
Describe how the utilization of data is changing and the emergence of the “Data Scientist” (or “a programmer who knows more statistics than a software engineer and more programming than a statistician”).
- How is data being used in innovative ways to ask new and interesting questions?
- What is Data Science?
- Data Science, Machine Learning, and AI: What is the difference?
- Data Analytics Life-cycle
- Discovery
- Harvesting
- Priming
- Exploratory Data Analysis
- Model Planning
- Model Building
- Validation
- Production Roll-out
Python for Data Analysis
Quickly introduce the Python programming language, its syntax, and the core libraries used to work with data from inside of Spark.
- Python Modules: Toolboxes
- Importing modules
- Listing modules
- Python Syntax and Structure
- Core programming language structure
- functions
- Comprehensions and syntactic sugar
- Python Data Science Libraries
- NumPy
- NumPy Arrays
- Pandas
- Python Dev Tools and Analytic Environments
- Jupyter
Overview of Course Project: Predicting Flight Delays
- What goes into making an airline run on time?
- Discussion of Requirements and Phases
- Building a Dataset: Extract, Transform, and Load
- Exploratory Data Analysis and Initial Model Building
- Enrichment: Utilize secondary data sources to provide additional context on what factors contribute to delay
- Streaming: Build an end-to-end application that is able to utilize machine learning to provide flight delay predictions
Spark SQL: Structured Data Fundamentals
Aggregating, repairing, normalizing, exploring, and visualizing data with Spark.
- Introduction to Spark: A General Engine for Large Scale Data Processing
- What is Spark?
- How is it used in practice?
- Building Datasets in Spark: Extract, Transform, and Load
- Configuring an environment for data analysis
- Importing data form external sources
- Inspecting data schema and structure
- Transforming data types, renaming columns, and managing values
- Spark: Explore and Visualize
- Calculating descriptive statistics and relationships
- Code categorical data
- Show how to represent a distribution in pictures (histograms and related charts)
- How does Spark integrate with the broader world of data visualization in Python?
SparkML: Machine Learning in Spark
- “The Machines are Coming”: Machine Learning and Artificial Intelligence
- What is machine learning, what makes it different than artificial intelligence?
- What are some ML techniques and how can they be used to solve business problems?
- Supervised versus unsupervised learning: what are the differences?
- Terminology and definitions
- Features and observations
- Labels
- Continuous and categorical features
- Machine Learning Algorithms and How They Work in Spark
- Classification and Regression: How do you build machine learning models to “make guesses” and “put things in buckets”
- Classification
- Regression
- Case Study: Predicting Flight Delays Using SparkML
- Clustering and Principal Components Analysis
- Time Series
Spark Streaming
What is so great about “streaming data” and how does Spark facilitate its analysis?
- Apache Kafka: A Streams Platform
- Spark Structured Streaming: Working with data that never ends
Case Study: Natural Language Processing
Show how machine learning techniques can be applied alongside feature engineering to solve complex problems.
- Introduce natural language processing, core constructs that can be used to work with human language.
- Explore computational models of human language for classification and clustering.
- Show how keyword extraction using NLP and data normalization can be used to locate patients with a specific condition or disease.
Case Study: Looking for Cancer
Utilize computer vision tools to analyze images for signs of malignant cells.
Predicting Flight Delays
Any given day, there are about 87,000 flights crossing the country, with about one-third being flights run by commercial carriers like American, Delta, United, or Southwest. At any given moment, 5,000 planes are in the skies above the United States in route to one of the 9,000 airports worldwide.
- Carriers work very hard to ensure that flights depart and arrive on time, but the logistical challenges in operating an airline are enormous.These include:
- ensuring there are sufficient numbers of pilots, ground crew, mechanics, and others to safely prepare and operate aircraft
- working with air traffic controllers and other safety regulators
- contending with weather
Frequently, despite every best effort, issues arise and flights are delayed. When that happens, what are the most important contributors and can such delays be predicted ahead of time with any precision?
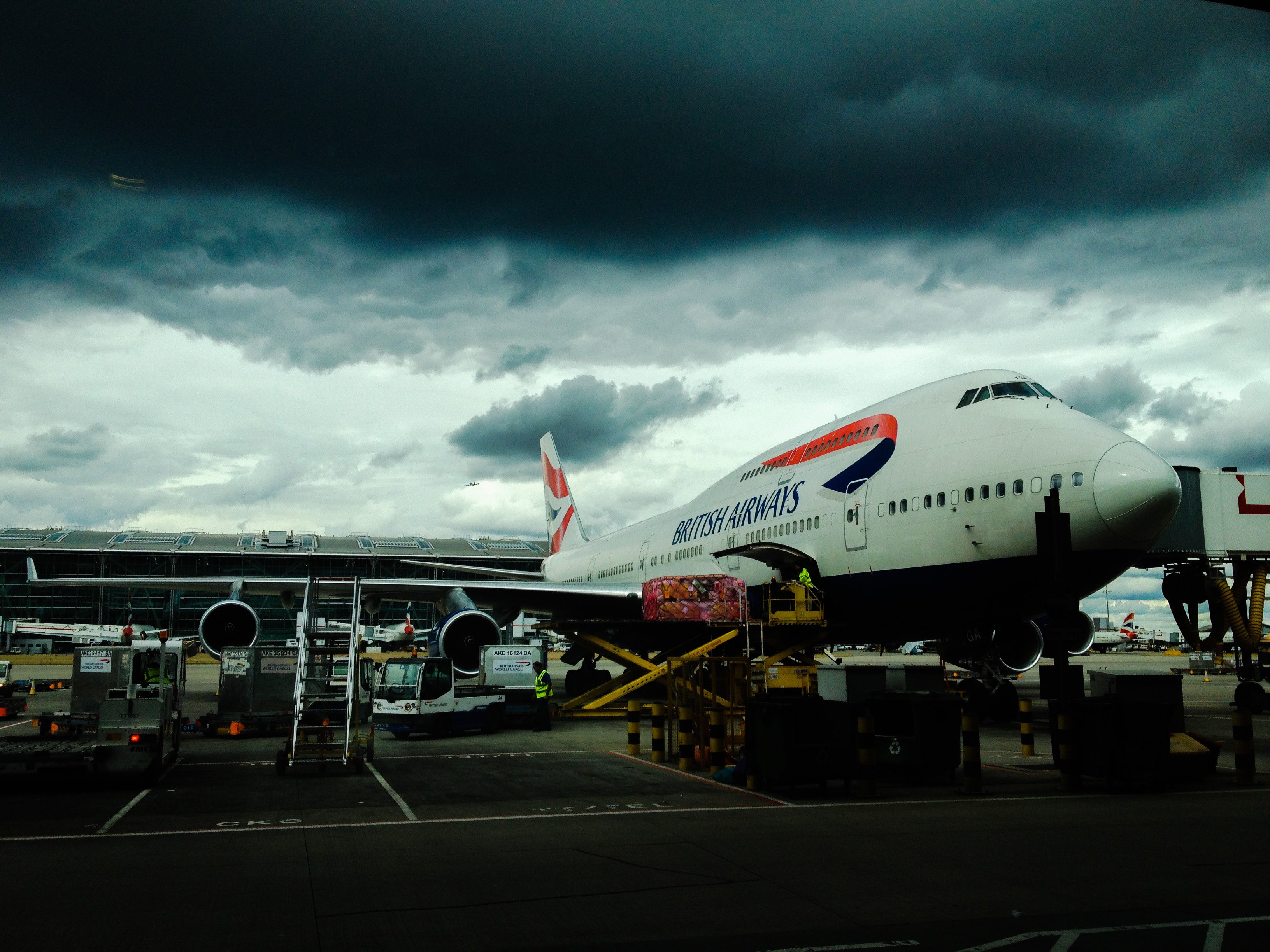
Building a Dataset: Extract, Transform, and Load (ETL)
Before you can have a machine learning model, first you have to have data. Most real-world data requires processing before it can be used, however, and requires a degree of transformation. ETL is a type of data integration that uses three steps -- extract, transform, and load -- to blend data from multiple sources.
During the process, data is taken from a source system (extracted), converted into a format that can be analyzed (transformed), and stored (loaded) into a destination system. In this step of the project, we will convert our dataset to a format which can efficiently analyzed.
Exploratory Data Analysis
The first step in any data analysis is to explore the raw data and try to get a feel for its organization and structure. This often involves creating summary (descriptive) statistics and visualizing the distribution and correlation of the variables in relation to one another. In this step of the project, we will use Python tools to dig into the flight delay data from the Department of Transportation.
Machine Learning
Machine learning can help us analyze datasets with thousands or millions of features and tell us which are most important to an outcome of interest. In this step of the project we will create machine learning models and assess their predictive accuracy.
Enrichment
Sometimes we need more context than the dataset at hand provides. In such cases, utilizing secondary data sources to provide additional context on what factors contribute to delay can shine more understanding of the problem. In this part of the project we will combine the primary flight delay data with publicly available data form the National Weather Service to see what role precipitation and temperature have on flight operations.
Streaming
Once a model exists, it can be used to work with new data. In this last step of the project, we will build an end-to-end application that is able to utilize machine learning to provide flight delay predictions.
Comments
Loading
No results found