AI in Medical Imaging
The promise of medical AI is a future where "the wisdom contained in the decisions made by nearly all clinicians and outcomes of billions of patients should inform the care of each patient." How do we get there?
Part 1: Improving Diagnosis and Care
Healthcare systems around the world are facing a multitude of pressures:
- Increases in the prevalence of complex chronic conditions, such as cardiovascular disease, which requires care from a team of specialists.
- A shift toward patient-centered precision care, where treatment is adjusted based on differences in anatomy or genetics.
- Changes to how healthcare is delivered caused by the COVID-19 pandemic where staff shortages, overwhelming patient loads, and significant delays in processing diagnostic exams are common.
These pressures come from a number of factors, not least among them:
- increased expectations from patients about what healthcare organizations are expected to deliver
- the fiscal reality of flat or declining resources available to pay for care
What might be done about these challenges? Are there resources available that can be used to improve patient outcomes and deliver care more efficiently?
Modern healthcare generates an enormous amount of information that is used for the diagnosis and treatment of disease. This includes "omics" data (genomics and proteomics), high resolution medical imaging, electronic healthcare record (EHR), individual genetic profiles, biosensors, and wearable devices. Could solutions about how to improve diagnosis and care be found in the data?
A Great Undertaking
Healthcare's flood of raw information holds tremendous potential in delivering a better future, especially when paired with AI. For patients it might mean improved diagnosis, streamlined/standardized image interpretation, and better guidance on treatment options for different disease stages. For clinicians, it might help eliminate variation in how patients are treated, streamline clinical workflows, and expand the availability of clinical expertise. A 2019 article published in the New England Journal of Medicine expresses a compelling vision:
What if every medical decision, whether made by an intensitivist or community health worker, was instantly reviewed by a team of relevant experts who provide guidance if the decision seemed amiss? Patients with newly diagnosed, uncomplicated hypertension would receive the medications that are known to be most effective rather than the one that is most familiar to the prescriber. Inadvertent overdoses and errors in prescribing would be largely eliminated. Patients with mysterious and rare ailments could be directed to renowned experts in the fields related to the suspected diagnosis.
While such a system might seem like a far-fetched fantasy, technologies already exist that can work together to provide many of the benefits of AI enabled healthcare. Consider:
A thirty-nine year old male presents to the emergency department (ED) with shortness of breath, dizziness, and fatigue. The patient has no previous history of heart or lung disease. He had been exercising when his fitness tracker detected an irregular heart beat/interval and prompted him to acquire an EKG where bradycardia (slow heart beat) was detected. The fitness tracker prompted the patient to seek treatment after he indicated that he felt fatigued and dizzy.
After acquiring an EKG (which showed normal heart rhythm), the attending physician also acquired a chest x-ray to screen for other conditions. The hospital's automated system for reading chest x-ray studies flagged evidence of early pneumonia consistent with COVID-19 and recommended a PCR based screening test be performed. The test returned positive for COVID-19 and the patient was admitted to the hospital for observation.
As we will see in this article, everything described in this anecdote can be accomplished with existing or near-future technology.
Bumps in the Road
But while the "promise" of AI is enormous, a future where "the wisdom contained in the decisions made by nearly all clinicians and outcomes of billions of patients should inform the care of each patient" is not a foregone conclusion. For nearly fifty years, experts have predicted that advances in computing would "[augment] and in some cases, largely replace the intellectual functions of the physician." Yet in 2021, that future is still over the horizon.
In some ways, the future has never seemed further away. There have been many "moonshot" projects, such as IBM's attempt to build the "Watson superdoctor," which have promised radical AI driven breakthroughs, only to spectacularly under-deliver. Will the next fifty years be a string of "almost there" solutions that have little impact on how care is provided?
Watson Superdoctor
Intended to bring AI into the clinic and to revolutionize diagnosis, Watson was a set of integrated AI services designed to streamline aspects of care such as image analysis, genetic analysis, and clinical-decision support. Perhaps the most ambitious project, however, was the initiative to create the "superdoctor."
Through the use of natural language processing, computer vision, and other advanced capabilities; Watson was able to take collections of patient symptoms and return a list of diagnoses, each annotated with a confidence level and links to the supporting medical literature. But while many groups have had success analyzing clinical text data and creating classification models from it, Watson was intended to do something more:
- read and analyze the medical literature
- extract relevant clinical insights
- apply those insights to new patients as a human physician would
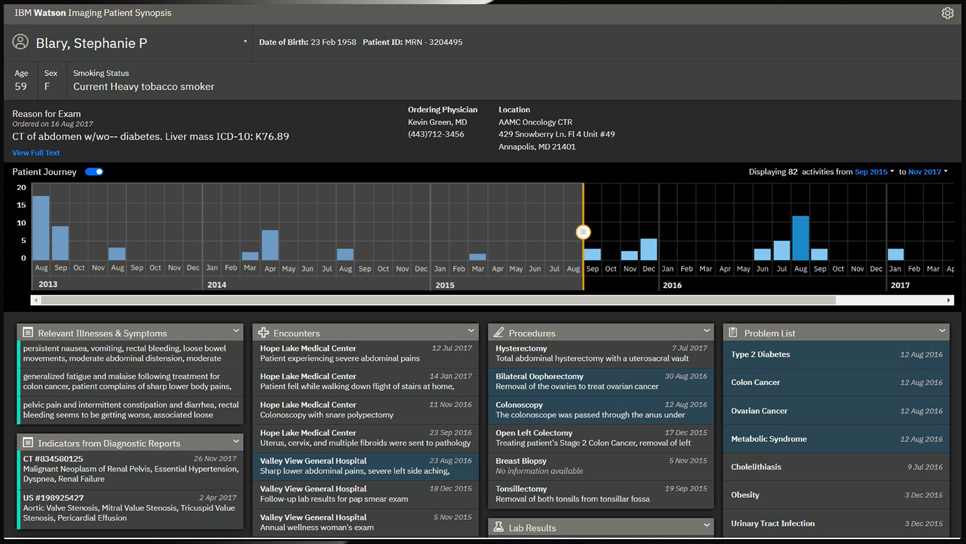
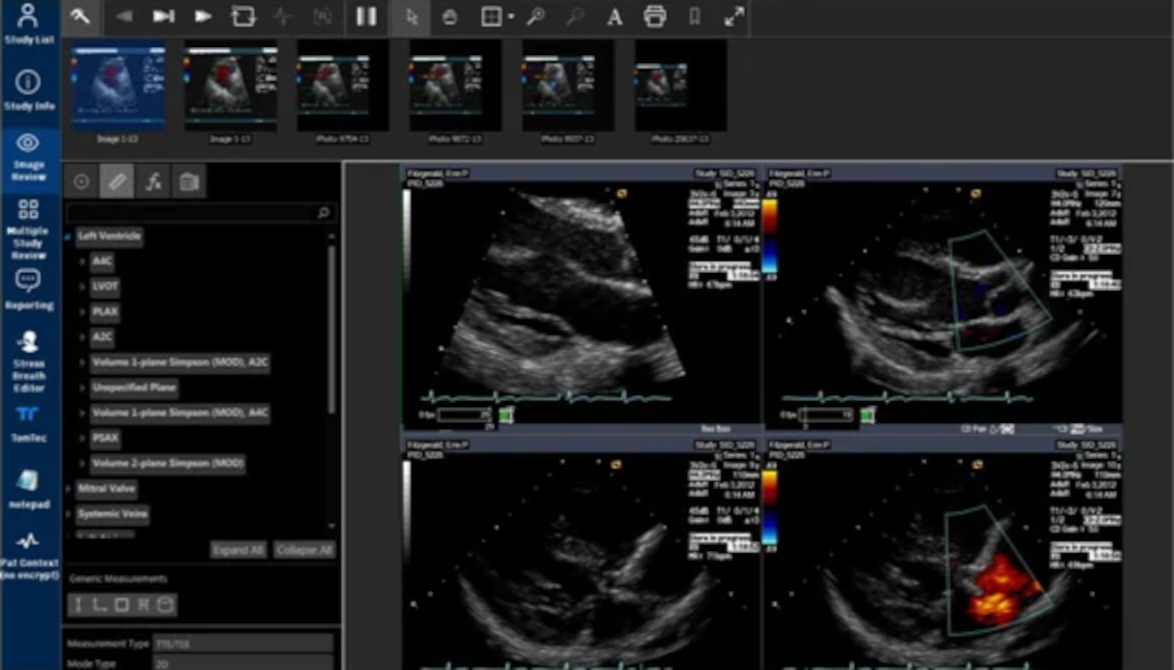
But after investing billions of dollars, Watson struggled to find relevance and traction in the marketplace. The project was plagued with misstarts and outright failures, and never produced a clinical product akin to the self-learning superdoctor originally envisioned. By 2019, IBM made the difficult decision to pivot to a new strategy, scale back ambitions, and lay off hundreds.
Ajay Royyuru, IBM's vice president of health care and life sciences research, explained in an article to IEEE Spectrum that part of IBM's decision was rooted in the lack of a business case. He said, "Diagnosis is not the place to go. That's something the experts do pretty well. It's a hard task, and no matter how well you do it with AI, it's not going to displace the expert practitioner."
Yet, diagnosis is central to the entire practice of medicine. Getting the right diagnosis provides an explanation of what is wrong and determines how a patient will be treated. An incorrect diagnosis can cause harm to patients by delaying appropriate care or providing harmful treatment, and they are all too common with medical errors accounting for millions of unnecessary procedures and tens of thousands of deaths per year. The National Academies of Sciences, Engineering, and Medicine calls the need to improve diagnosis a "moral, professional, and public health imperative."
So if not a superdoctor, what role could AI play?
Driving AI Adoption
While systems that aim for "general intelligence" have had mixed results, specific applications of Narrow AI have found their way into clinical practice. In January 2017, the FDA granted 510(k) approval for Arterys Cardio DL, a web accessible image processing system for viewing and quantifying MRI images. Since then, approval for scores of additional models has also been granted.
Large healthcare systems have used machine learning models for a number of applications such as identifying patients who may require more intensive care, accurately evaluating knee MRIs to prioritize which patients may require treatment, and correctly diagnosing pneumonia from x-rays. Products have also started to emerge that help streamline data entry and optimize clinical workflows.
Outside of major clinical centers and large academic institutions, however, adoption of AI has been slow. Getting access to high quality and representative data for training machine learning models is extremely difficult, and small organizations do not have the researchers, technologists, and infrastructure needed to train and deploy AI systems. But though adoption has been slow, other trends in technology are helping to drive advances and there are hopeful signs on the horizon:
- The inclusion of regulated medical technology into consumer devices, such as the Apple Watch, now allows for earlier capture of diagnostic data and AI facilitated assessment. Because they are on and monitoring (basically) all the time, such devices offer the potential for much earlier detection of disease and can help prompt users to seek medical attention or to log details about an episode that may prove important in later diagnosis and treatment.
- The broad availability of mobile phones and other small-form computing devices have enabled portable imaging hardware, such as the Remidio Fundus on Phone and Butterfly Ultrasound probe. Smaller and cheaper than standalone systems, these new devices allow for diagnostic screening to be expanded in rural and other under-served communities. Paired with cloud based AI (that isn't tethered to a specific healthcare provider) to read the images -- which show comparable levels of accuracy with trained specialists -- the combination of technologies can greatly expand access to care.
- The emergence of interfaces that allow AI solutions to integrate with other hospital systems and to "work alongside" healthcare professionals. Such "virtual specialists" help emergency room physicians, general practitioners, and other care providers extend their clinical expertise, perform triage, and improve the timeliness of treatment during off-hours or emergencies if a specialist is not available.
In the remainder of this article, we will look at these examples in detail.
Blending Medical and Consumer Devices
Note: While not strictly medical imaging modalities, ECG and other measurement techniques not intended to create images such as EEG and MEG are closely related technologies often incorporated into medical imaging data and AI solutions. They are part of the DICOM standard supplement 30, and many tools provide support for retrieving and working with them alongside other forms of imaging data.
In early 2021, Apple released version 7.3 of their Apple Watch operating system with support for an EKG app capable of analyzing heart arrhythmias.
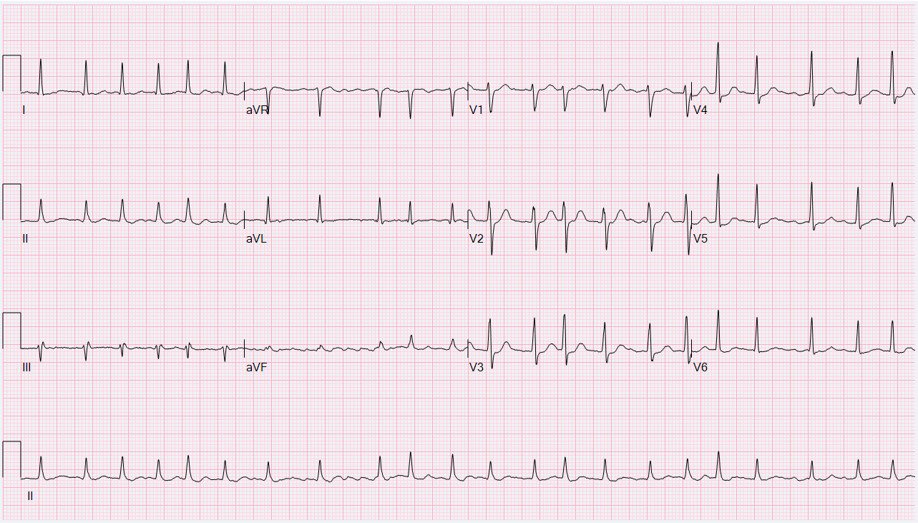
Using an electrical sensor built into the digital crown and the back crystal, the watch is able to record a single lead EKG which is analyzed to determine if the heart rate is normal, atrial fibrillation (AF), or AF with high heart rate. It will then prompt the user to record any symptoms such as dizziness, pounding heartbeat, or fatigue. The waveform, date, time, and symptoms can then be sent to a physician.
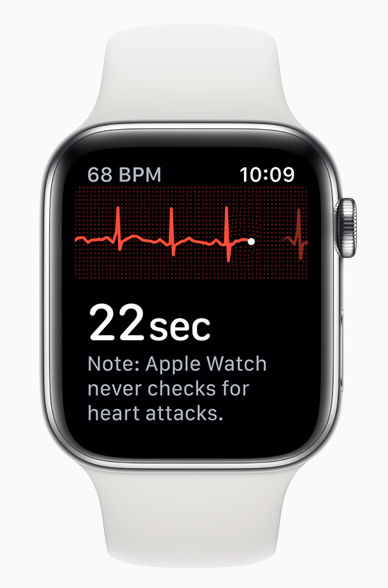
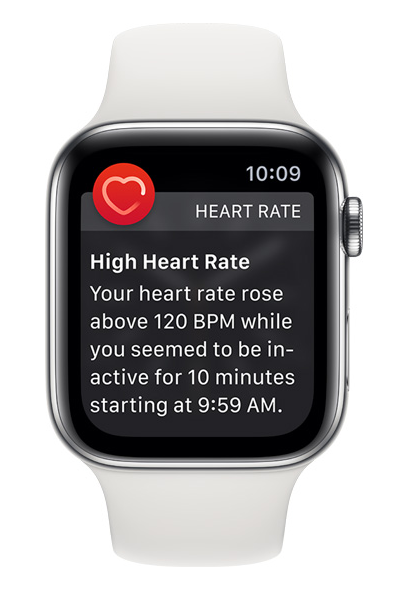
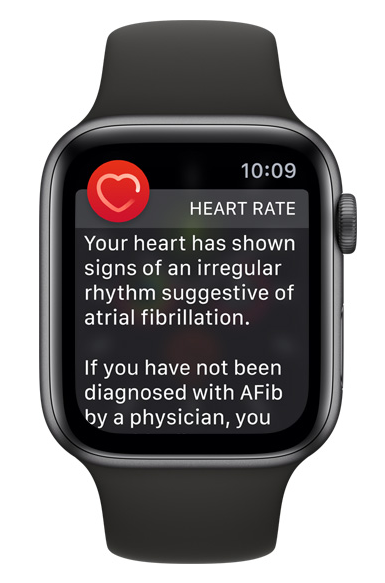
Wearable computers and cheap sensors now allow for the capture of clinical data almost anywhere; and AI offers the potential to impact how patients interact with the medical system. Instead of a patient suffering some type of episode and then seeking care from her physician, AI devices (such as the watch) are able to monitor streams of physiologic data and check for irregularities. They can screen for signs of disease, such as a high heart rate, and notify the user/patient of the abnormality.
Historically, atrial fibrillation and other arrhythmias have been difficult to diagnose due to their intermittent nature and may not be recognized until they are serious and frequent enough to cause other symptoms such as shortness of breath or dizziness. Blurring the lines between medical and consumer device, though, the Apple Watch is basically on and recording all the time. It's in a position to capture diagnostic evidence of an irregularity far earlier than more traditional screening tools such as EKG or Holter, and incorporates technology that is able to prompt users to take action on anomalies.
The type of action can vary based on the duration and severity of irregular heartbeat. The watch might ask for additional information to describe what type of symptoms may have accompanied the episode, for example, or it might encourage the user to contact caregivers. The collection of such multi-channel data -- sensor, physiological stream, and user-reported symptoms -- provides a way to greatly improve AI models (basically implementing a form of human in the loop), but the implications could go beyond that. With consent, the watch might also be able to initiate contact with the emergency system if the patient/user is incapacitated and unable to do so herself.
Though Apple does not release sales numbers, industry analysts have estimated that more than one-hundred million Apple watches have been sold since its release in 2015, with about half of those devices supporting the EKG feature and passive pulse monitoring. This makes Apple Watch one of the most widely available medical devices on the market. Because atrial fibrillation is not always associated with symptoms, many Apple Watch owners may have atrial fibrillation and not know it. The combination of early detection, automated assessment, and user interaction provides a powerful tool for the understanding and treatment of arrhythmias and a template for gaining insight into other diseases, even for patients that are not yet aware of a condition.
Expanding Treatment
The benefits of such technologies are not solely related to luxury consumer devices. In 2016, Google Health published the results of a multi-year study to assess the use of AI in the developing world.
Diabetic retinopathy is a progressive disease that is common amongst those with diabetes. If detected early, it can be treated successfully; if not detected, it leads to irreversible blindness. One of the best ways to detect retinopathy is to acquire images of the back of the eye and have them read by specialist physicians. Unfortunately, such specialists are not available in many parts of the world where diabetes is present and early disease can be missed when read by practitioners without specialist training.
For rural communities, high prevalence of diabetic retinopathy often is an issue of poor infrastructure and lack of care. A rural patient may have ailing eyesight and an advanced stage of diabetic retinopathy, but not know they are diabetic. They might not have access to the equipment needed to acquire the images or an ophthalmologist specializing in retinopathy available to read the studies. To successfully treat retinopathy, however, screening needs to happen early on, while a patient's vision is still good. Such "specialty needs" are just the type of tasks that current models are able to perform with high accuracy.
But having a trained model isn't the only challenge, acquiring a retinal scan isn't quite as simple as pointing a camera into the eye and pressing the shutter.
Luckily, the same trends which allow for EKG systems to be built into watches also enable high fidelity optical imaging from smartphones. It's entirely possible to use smart-phones with indirect condenser lenses on patients with dilated pupils, for example, and the same "do it yourself" philosophy is leading to portable devices like the Remidio Fundus-On-Phone, which provides traditional optics but relies on the phone's camera to acquire the image.
Since they are portable and new operators can be trained quickly, such "Fundus On Phone" systems offer a way to increase screening in rural communities without dedicated clinics. Because the smart-phones used to capture the image are connected to the cellular network, the images can then be sent to cloud based tools for analysis. Once in the cloud, a "Neural Net" can assess the image for hemorrhaging, nerve tissue damage, swelling, and other markers of diabetic retinopathy.
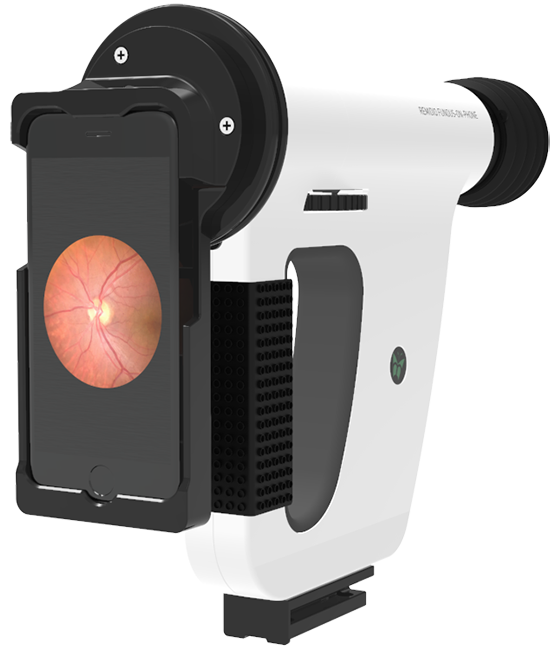
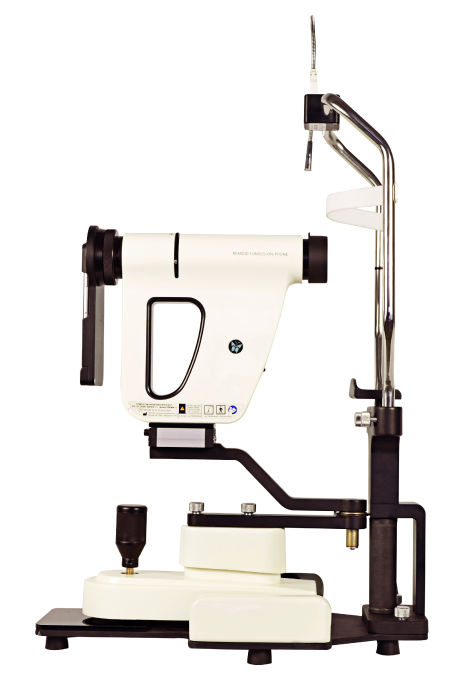
The AI models created by Google were generated by "training" on retinal scans that have been "labeled" by ophthalmologists on a scale from zero (no disease) to five (extreme signs present), and made use of cutting edge neural network architectures in the hope patterns recognized by the model would be similar to the signs of diabetic retinopathy an ophthalmologist would identify.
Augmenting Human Intelligence
While AI models are capable of identifying disease with roughly the same accuracy as trained specialists, they are also extremely valuable in helping care providers (both specialists and generalists) increase the accuracy and confidence of their diagnosis.
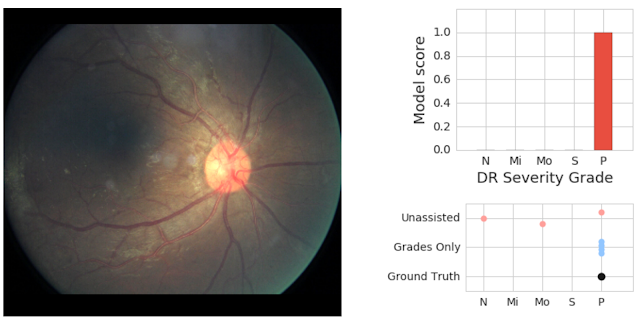
In a follow-up study published in 2018, Google Health demonstrated an important point in how AI systems can be used within the clinical environment. Through the use of AI as an initial screening tool alongside physician assessments of the same images, model predictions can be effective in helping physicians catch pathology they might have otherwise missed. The retinal image shown above shows an image with severe disease, a finding missed by two of three doctors who graded it without seeing the prediction of the model.
Similarly, physicians and AI can work together to provide more accurate assessments than either individually. The image below shows a retinal scan labeled as having moderate retinopathy. Without assistance, two of the three care providers reading the scan would have diagnosed it as "no disease," and the patient might miss needed care from a specialist.
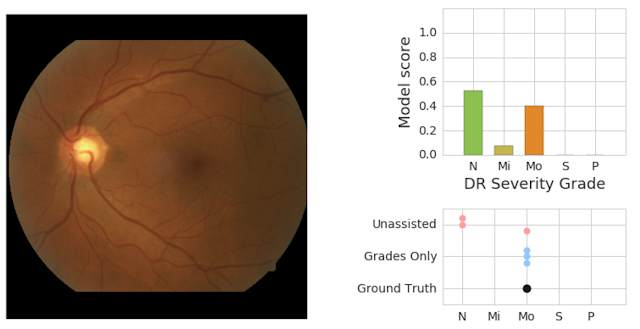
Virtual Specialists
Managing the care of patients during off hours (such as the middle of the night, on weekends, or holidays) has always been a challenge. In a busy emergency room, patients may wait hours for treatment due to lack of a care provider capable of reading a scan or interpreting a lab. In extreme cases, care may even need to be delayed hours or days until a specialist can be located.
In such circumstances, there is an opportunity for AI to help expand the availability of clinical expertise. AI might be used to provide an initial reading of a patient scan so that treatment can commence, for example, followed up by reading from a specialist later. Or it might empower nurses to take on more demanding tasks (such as those currently performed by physicians), allow primary care doctors to assume some of the roles of specialists, or enable specialists to devote additional time with patients requiring more of their expertise.
What has always been a challenge has become a crisis due to the COVID-19 global pandemic. Almost overnight, hospital systems have had to cope with multiple simultaneous misfortunes:
- overwhelming patient loads due to COVID that consume available resources and disrupt other care within the hospital
- lack of PCR diagnostic test kits that could be used to reliably confirm diagnosis
- a new infectious illness that has caused a crushing demand for specialized acute care while also resulting in a reduction of as much as 60% for routine office visits, resulting in losses in the hundreds of billions of dollars for care providers
These needs have caused a number of organizations, including Royal Bolton Hospital in the United Kingdom and the UC San Diego Health System, to turn to AI systems to help them manage the COVID-19 induced shortages and to fast track promising new technologies.
X-Ray COVID Screening
Using AI to help screen for COVID-19 cases has been amongst the most ambitious of these initiatives. Research published early in the pandemic provided evidence that serious COVID cases could be detected through the use of chest x-rays (CXR). This evidence elicited a great deal of enthusiasm amongst the medical imaging and AI communities, resulting in the launch of several public initiatives to create machine learning models; and causing a small arms race amongst research groups, startups, and healthcare companies to develop AI based tools. Given the early shortage of testing and delays in receiving PCR results, CXR looked like a promising technology for helping physicians to triage patients within minutes rather than hours or days.
The ability to rapidly prototype and assess new COVID screening models was possible because of well prepared initiatives making datasets publicly available for researchers to use for development. Because they are inexpensive and easy to acquire, CXR is amongst the first diagnostic tests ordered for patients who present with signs of heart or lung disease. They are a ubiquitous part of care and there are a tremendous number of imaging series stored in hospital PACS along with radiological reports. Recognizing that these scans represented an untapped resource, in 2017 the United States National Institutes of Health (NIH) released a massive dataset of CXR studies containing 108,948 images from 32,717 unique patients. Additionally, they released a set of labels describing fifteen unique conditions, including pnuemonia.
Using these datasets, a number of Deep Learning models have emerged that are capable of screening for lung disorders with high accuracy. These models are able to encode features of disease and detect specific conditions, even when other pathologies may be present, and have shown promise for building systems capable of aiding with triage.
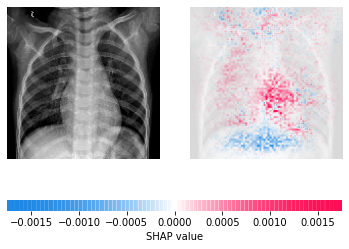
COVID researchers have been able to build upon this work to create new models capable of detecting infections with high accuracy and differentiating between COVID-19 and non-COVID pneumonia. They have combined the data available from ChestX-ray8 (and other databases such as ChestX-ray14) with COVID-19 scans to create new public datasets. They have applied the lessons learned from previous work and not only created models capable of screening for disease but also prognostic models to try and determine risk for severe outcomes such as death, need for ICU admission, or ventilation.
As of March 2021, sixty two research papers had been published with many additional studies available in pre-print status. And while no single paper has yet reported methodology ready for direct clinical implementation (showing a fully reproducible method, end to end best practice adherence, and external validation), there is "fantastic promise for applying machine learning methods to COVID-19 for improving the accuracy of diagnosis ... while also providing valuable insight for prognostication of patient outcomes."
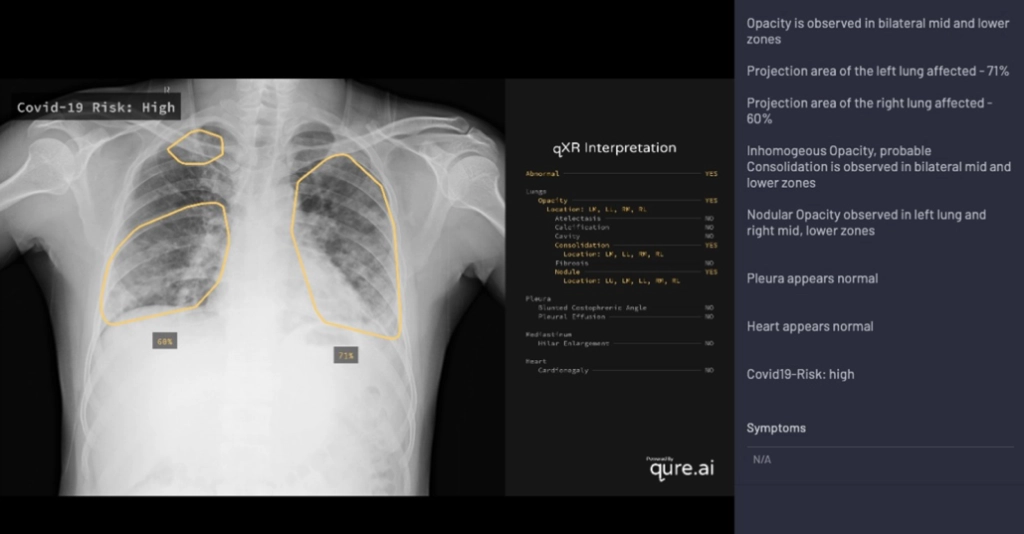
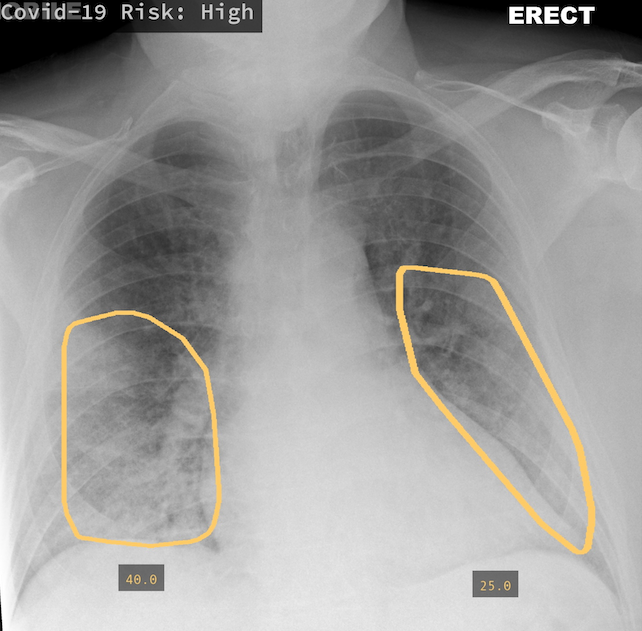
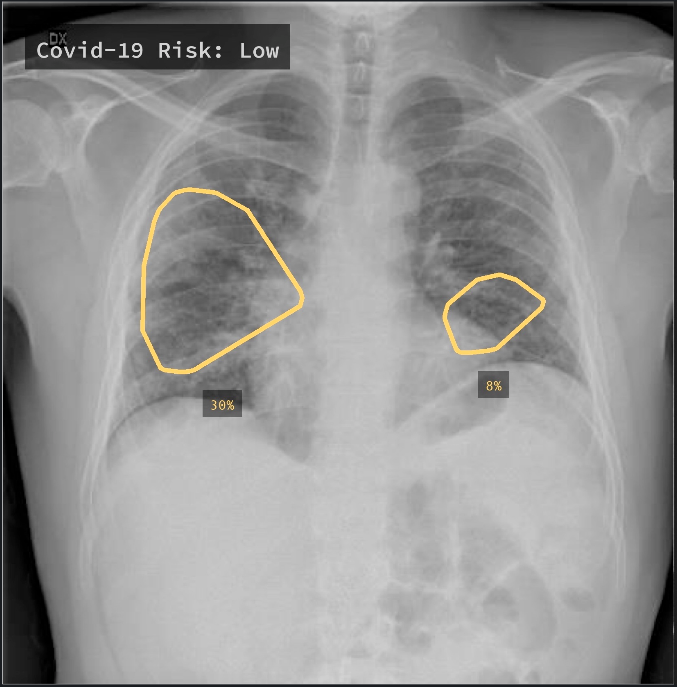
Alongside new research, AI driven screening tools have started to find their way into products. One company, Qure.ai, was amongst the first to release support for COVID screening as part of qXR, an automated X-Ray Interpretation platform.
As with the researchers using ChestX-ray8 and other publicly available datasets, Qure.ai was able to rapidly prototype a model by leveraging an existing set of images (though in this case a private database used for product development). By the end of March 2020, just as COVID-19 was being declared a pandemic by the World Health Organization, the first iteration of qXR's model became available for testing.
Trained on 2.5 million CXR studies, the model accepts features from qXR and considers findings indicative of COVID such as Opacities
and Consolidation
as well as those contra-indicative of COVID to generate a risk score of infection. Displayed prominently in the UI alongside a detailed report of the model's assessment, the score is intended to help front-line physicians or other care providers triage patients and provide guidance on treatment. In addition to the risk score, the tool also creates overlays to show where in the lungs signs of COVID may be visible, and if multiple images were acquired, is capable of tracking changes over time.
From the time of its release, Qure.ai has seen significant interest in the qXR COVID triage tool. Under enormous pressure because of staff shortages, overwhelming patient loads, and delays in processing PCR tests; hospitals have been hungry for solutions that can help make a preliminary diagnosis more quickly.
At Royal Bolton Hospital, Rizwan Malik (one of the lead radiologists for the hospital) had been assessing qXD as a tool to help with training of new fellows prior to the pandemic. Once COVID hit, though, he saw great promise in the technology and adjusted his proposal to use it for triage. qXD was moved quickly to a trial as a first line screening tool for COVID patients, as reported by the MIT Technology Review:
Normally the updates to both the tool and the trial design would have initiated a whole new approval process. But ... the hospital green-lighted the adjusted proposal immediately. "The medical director basically said, 'Well do you know what? If you think it's good enough, crack on and do it ... We'll deal with all the rest of it after the event.'"
Qure.ai has seen similar interests from hospitals in North and South America, Europe, Asia, and Africa. qXR has been adopted for screening and triage at hospitals in India and Mexico. A hospital in Milan, Italy deployed qXR to monitor patients and to evaluate response to treatment. In parts of Karachi, India qXR powered mobile vans were used across multiple clinics to identify potential carriers of COVID-19.
Demand for similar products from other companies, such as Lunit's Insight CXR, has also been strong since the beginning of the pandemic. This was a distinct change from prior to COVID, where AI solutions struggled to gain traction. And also a distinct change from how IBM's Watson was received. Pierre Durand, a radiologist in France who founded a teleradiology practice called Vizyon, shared his experience in trying to broker sales for AI startups (via the MIT Technology Review):
"Customers were interested in artificial-intelligence applications for imaging," Durand says, "but they could not find the right place for it in their clinical setup" The onset of COVID changed that.
Virtual Assistants Not Superdoctors
While COVID-19 may have accelerated the adoption of AI solutions such as qXR and LUnit Insight, it didn't create the tools. Both qXR and LUnit were approved prior to the crisis by the European Union's health and safety agency. Instead, the pandemic highlighted how such "virtual assistants" could help to extend the reach of clinicians.
Nearly all types of currently approved AI products represent this type of solution. The very first FDA approved AI device, Cardio DL, is itself marketed as a virtual assistant and much of Arterys' product development work focuses on creating a platform to manage such assistants.
New products that have been released since Cardio DL such as Lung AI, Chest|MSK AI, and Neuro AI provide similar diagnostic support as qXR and LUnit, but couple with workflow automation that comes via integration with the Radiology Information System (RIS), PACS, electronic medical record (EMR), and dictation system. They process DICOM data to generate labels, annotations, heatmaps, and bounding boxes around regions of suspected illness. They then push those labels into the medical IT infrastructure to help prioritize the review of studies that may have abnormal findings and emphasize aspects of the study that may be concerning.
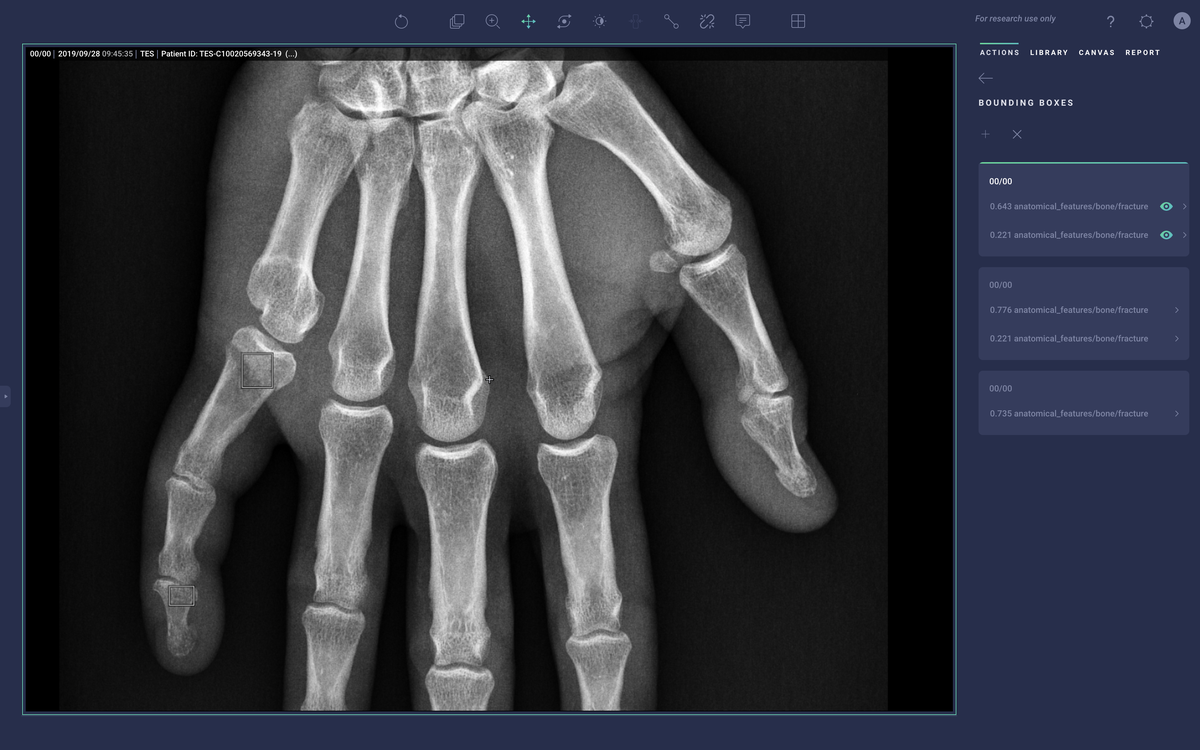
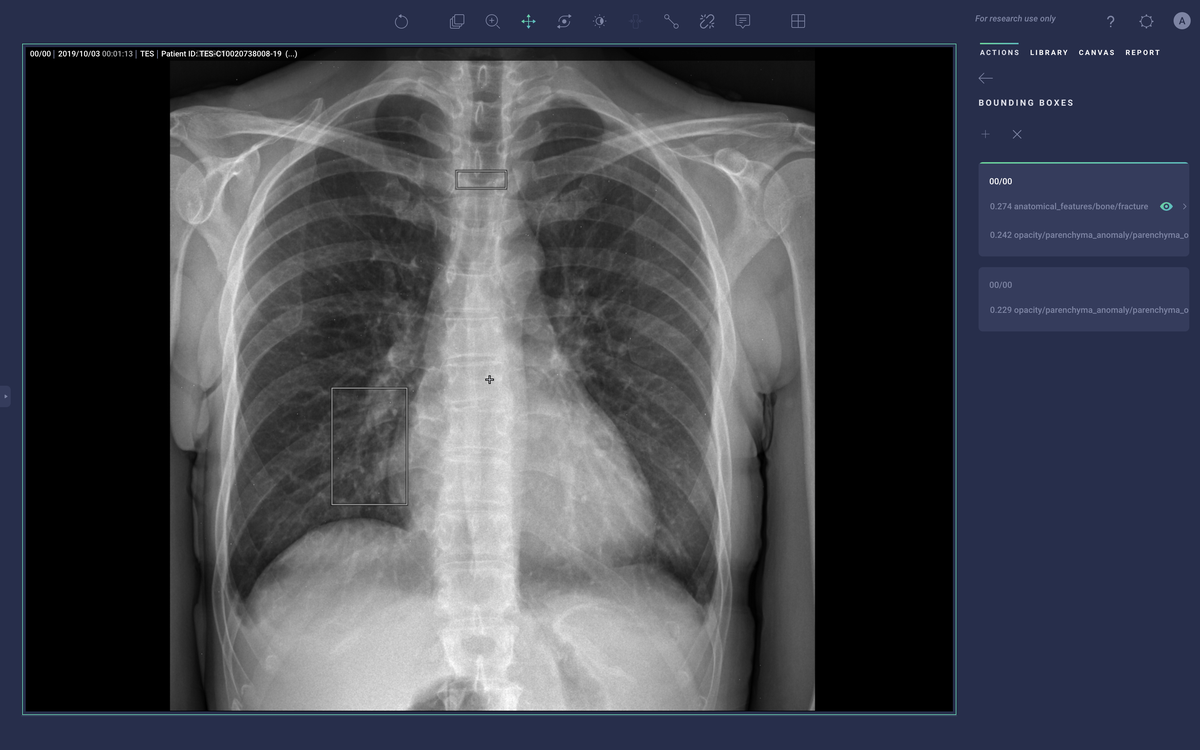
Converging on Solutions
While there are still significant challenges to widespread adoption of AI within healthcare -- the availability of high-quality data, issues related to bias and generalization, ensuring that healthcare disparities for disadvantaged populations don't become reflected within models, and challenges in regulation and oversight; just to name a few -- progress is being made in using AI to improve the diagnosis of disease and care of patients.
- The continued incorporation of medical sensors and technology into personal electronic device, AI facilitated assessment, and interactive interfaces provide opportunities for detecting diseases earlier in their course and prompting users to seek treatment.
- Mobile computing platforms, smaller and cheaper image acquisition modalities, and cloud computing allows for AI based disease screening even in the absence of permanent medical facilities. This is capable of expanding access to care for rural and underserved populations.
- The adoption of "Virtual Assistants" which can be used to help provide guidance on diagnosis and treatment enable care providers to augment their clinical expertise and interpret studies (even if specialists are not available), streamline the delivery of care, and triage patients.
If we return to the clinical anecdote from earlier in this article, we can see that AI has a place in clinical practice today. Using technologies such as the Apple Watch, it is possible to detect arrhythmias, prompt for additional information to determine their presence, and encourage individuals to seek treatment. AI assistants (such as qXR, LUnit, and Chest|MSK AIor) are capable of screening CXR imaging studies and prompting physicians to further explore abnormal findings. As the healthcare industry continues to grapple with significant challenges, data and AI provide powerful tools to improve care. AI can help patients receive the best possible care, tailored to their needs and circumstances, in the most efficient manner possible.
Comments
Loading
No results found