Detecting Lung Cancer with Artificial Intelligence (Part 1): Technical Difficulties
Lung cancer is one of the world’s most prevalent, deadly, and financially costly cancers. It accounts for 2.21 million deaths per year worldwide and is the leading cause of cancer mortality. Just within the United States, there there are hundreds of thousands of cases, of which a significant number die. In 2018, for example, there were 218,000 lung cancer diagnoses of which 142,080 cases were fatal (65% mortality) despite significant efforts and expenditures for detection and treatment. In 2020 alone, $23.8 billion USD in claims were made for lung cancer treatment accounting for nearly 14% of the total ($174 billion) spent on cancer treatment that year.
Early detection, diagnosis, and treatment are critical to reducing mortality and cost. While significant progress has been made in reducing the death rate of other cancers, the five-year survival rate of lung cancer (18.6%) is much lower than colorectal (64.5%), breast (89.6%), and prostate (98.2%). Yet, if detected early, the five-year survival rate rises to 56%. Unfortunately, only 16% of lung cancer cases are diagnosed at an early stage. Worse, nearly two-thirds of lung cancer patients are diagnosed during advanced stages of cancer when curative treatment is no longer possible. If lung cancer could be detected earlier, while the disease is still located within the lungs and has not spread to other parts of the body, there is hope that we could significantly reduce the death rate.
Why is early detection such a challenge and what are the technical issues which must be overcome? There are many reasons:
- The most common method of screening for lung nodules, chest x-rays, is not the most sensitive for detecting early-stage growths. In a study from the United Kingdom, is estimated that up to 20% of lung cancers may be missed if using X-Ray alone.
- More sensitive screening methods, such as low-dose computed tomography (CT), which are able to more accurately depict anatomical structures, are not perfect. While about 90% of presumed mistakes occur on chest x-rays, 5% occur when reading CT scans (with the remaining 5% on other imaging studies).
- There is a high degree of "observer error" where the lesion is missed because of how the scan was read or the tumor went unrecognized.
These points lead to a number of questions. Can AI help to provide a "second pair of eyes" for detecting tumors more accurately and earlier in their progression? What type of impact might a successful AI system realistically have on lung cancer treatment? Is this the type of problem that AI is capable of tackling?
In this series, we'll attempt to answer these points. First, we'll look at the technical challenges of early detection and survey some of the progress being made. In Part 2, we'll try to place how these challenges in the context of how lung cancer is currently treated. In Part 3, we'll take the conclusions of that analysis and use them to describe the shape of an AI solution.
Technical Difficulties
The primary difficulty of detecting cancer in x-rays and other radiology scans can be summed up succinctly: identifying candidate nodules, especially if they are in their early stages, is a very difficult task.
- Many of the first-line tools used for cancer screening (such as x-ray) are utilized because they are ubiquitous and inexpensive, rather than being ideal for the task.
- The work must be performed by highly trained sub-specialty physicians, requires painstaking attention to detail over long periods, and is dominated by images where no cancer is present.
- Unfortunately, more sensitive methods such as low-dose CT scans also suffer from limitations and challenges.
Analyzing Chest X-Rays
Though imperfect, chest x-rays are used as the first screening for lung cancer because of their ubiquity and low cost. When a patient presents in an emergency room or at a clinic with complaints about chest pain, fatigue, or shortness of breath; one of the first things the physician will order is a chest x-ray.
In a single image, x-rays make it possible to review the heart, lungs, blood vessels, airways, and bones of the chest and spine. They are easy to acquire, low cost, and can be used to rule out a host of potential conditions which may be causing the symptoms prompting the patient to seek treatment. Unfortunately, using x-rays for cancer detection has significant limitations:
- Because x-rays are a form of projectional radiography, structures in the foreground or background can make it difficult to see a cancer nodule. This might happen because the growth is covered by a dense structure (such as a rib) or is too small to be effectively visualized on the scan. Small tumors with poorly defined edges may be difficult to recognize because they don't result in a large enough opacity to be identified.
- Radiologists may ignore subtle densities thinking that they might be due to image artifacts (maybe due to motion) or other technical defects. Or they might misclassify the tumor, labeling it as benign when it is really cancerous.
- A phenomenon known as “satisfaction of search” occurs when the reviewer has detected one condition and effectively "loses interest" in the scans. As in many diseases, it is possible for a patient with lung cancer to have more than one disease (perhaps there is pleural effusion present well) and the consequences of skipping over a small nodule can be significant.
The images in the panels below highlight some of these difficulties. Example 1 shows the chest X-ray of a 62-year-old man with a tumor located in his upper right lung. The tumor is partially obscured by the collar bone and is difficult to see. When analyzed by a group of twelve radiologists, three members of the panel missed the tumor as part of their review. Though they recognized the presence of the tumor during a second review (more detail about that below).
Example 1: 62 year old man with adenocarcinoma
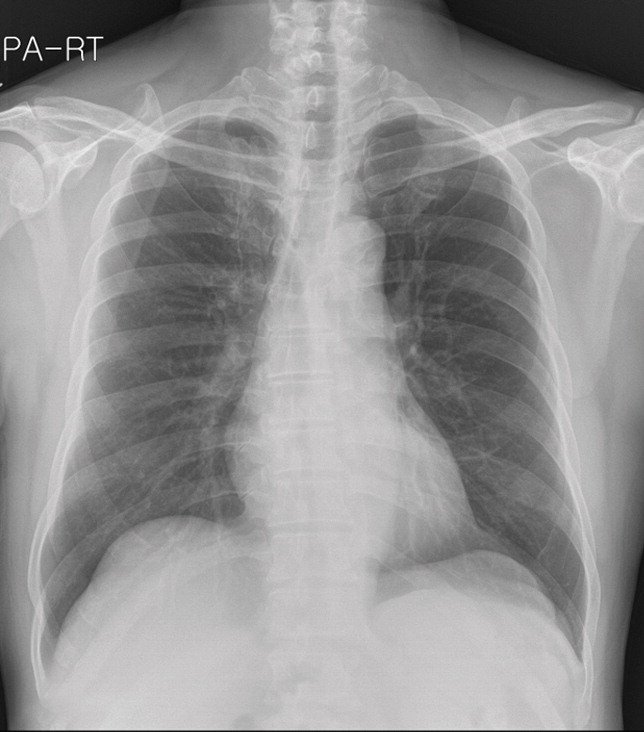
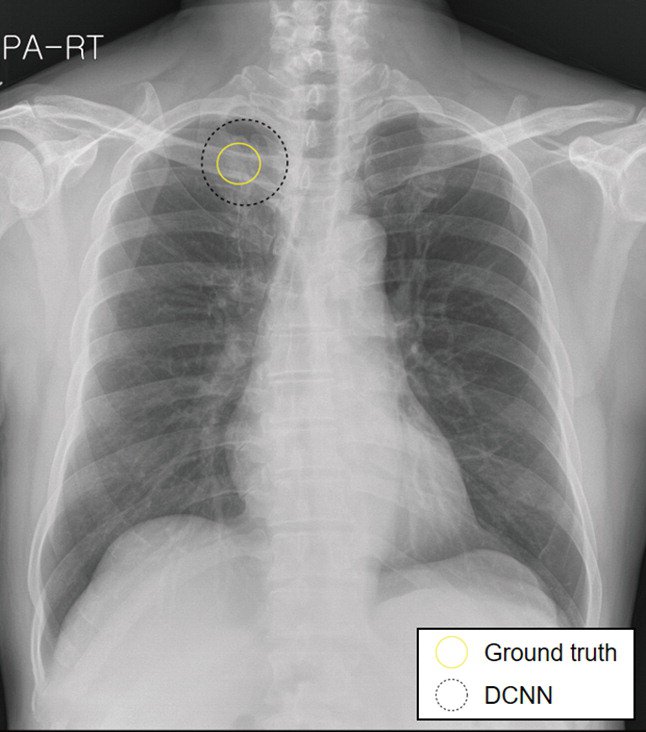
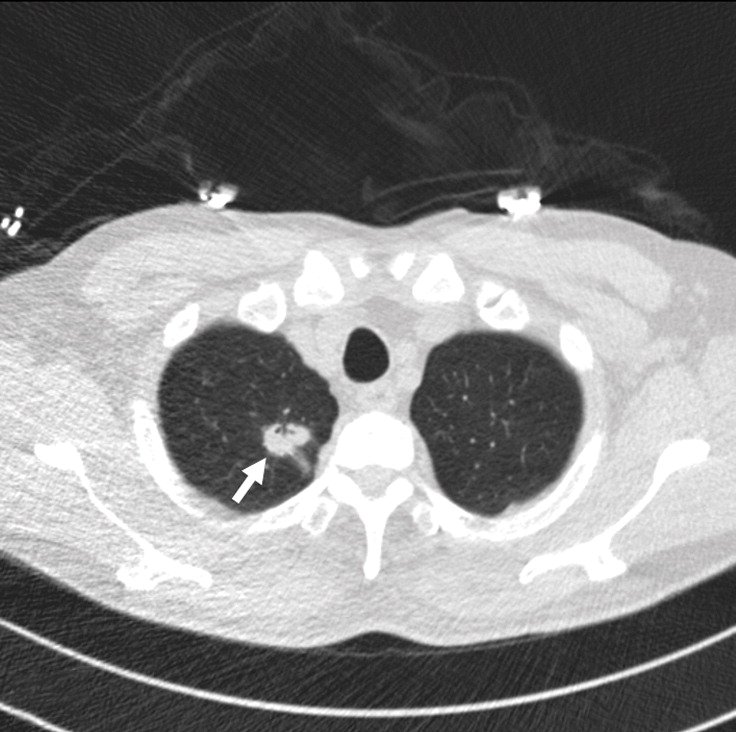
CT Is Better, But Not Perfect
Because of the limitations of x-ray, in 2013, the US Preventive Services Task Force formally recommended low-dose CT as an option for high-risk populations such as former smokers. The use of CT is better for many reasons:
- Effectively a narrow slice through a patient's torso, CT provides two-dimensional images without superposition (the overlapping of structures that happens in projectional modalities) at greater contrast and higher spatial resolution. These differences make it much easier to review and find small nodules that would otherwise appear invisible on an x-ray.
- There are a number of technical factors, such as the "thickness" of slices which can be adjusted to ensure that all tumors within the lung space are visible. Thin slicing (where the thickness is less than 1.25mm), in particular, has been shown as a good way to avoid errors; but at the cost of forcing radiologists to review more images.
Though far more accurate for cancer screening, many of the issues which prevent early detection in chest x-rays are also present in CT (though at a lower rate). Radiologists inaccurately interpret results, fail to recognize important findings and give up too early (satisfaction of search). What this means is that CT as a tool for early detection has mixed results: small, centrally located nodules with poorly defined borders remain the most likely to be missed or are at risk of incorrectly being labeled as benign.
In addition to cases where nodules are missed (or scans are incorrectly read), there is a further concern: inter-grader variability. Even when radiologists agree about the presence of nodules, differences in how they are classified are relatively common. In a 2017 study looking at how Lung-RADS grades (a model used to determine malignancy risk) are assigned, disagreement occurred in 29% of all readings. These variations included "significant" differences in 8% of cases, with the potential to impact treatment recommendations. Inter-observer variability has even been seen among radiologists in a single institution.
Example 2 shows an example CT scan of a 63-year-old with some of the characteristics described above. The nodule in the scan is small and close to a group of vessels of the upper left lung that partially block it from view, which caused the radiologist reading the scan to miss the tumor. The tumor was detected using a chest x-ray two years later.
Example 2: 63 year old patient with a cancerous lung nodule
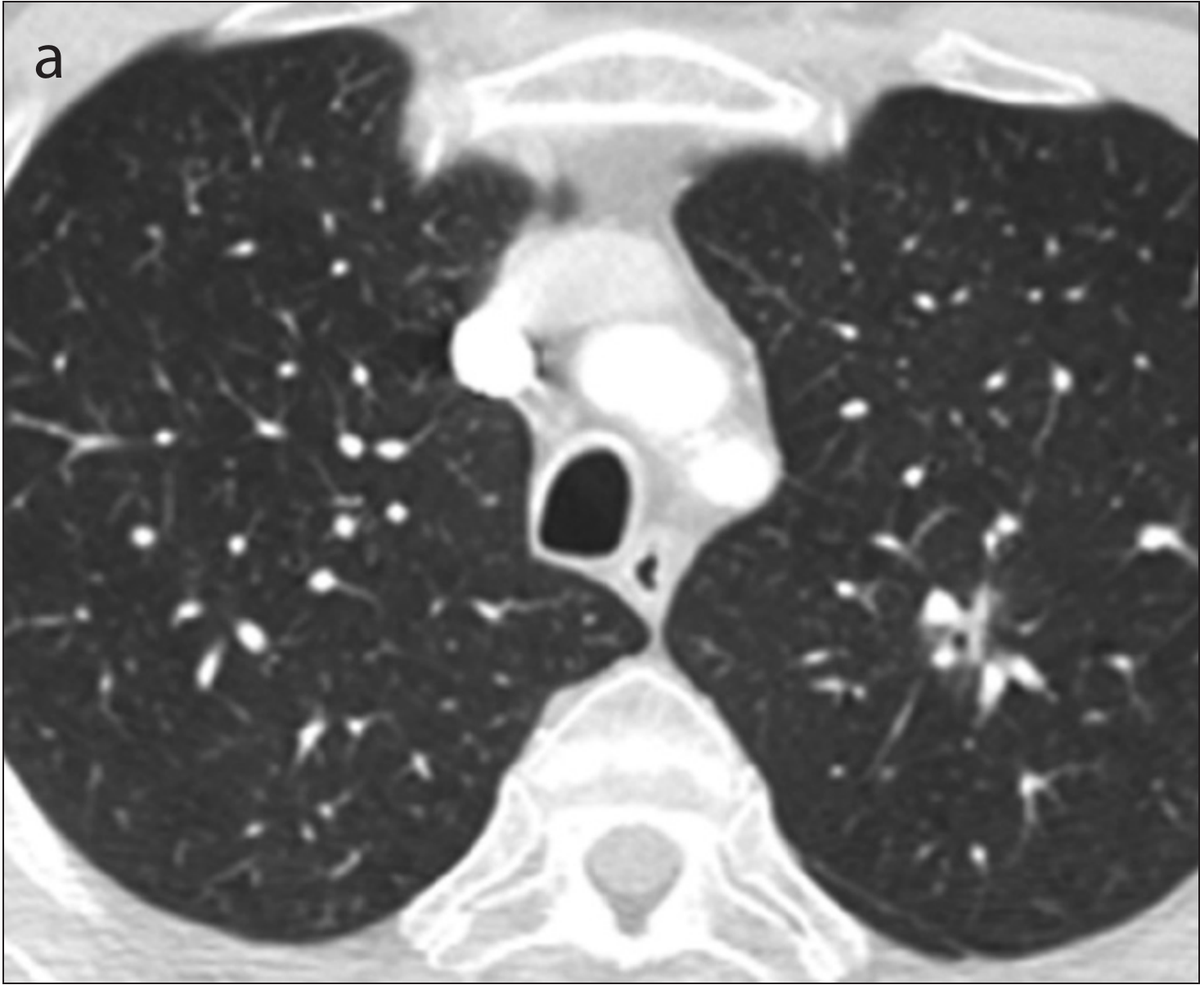
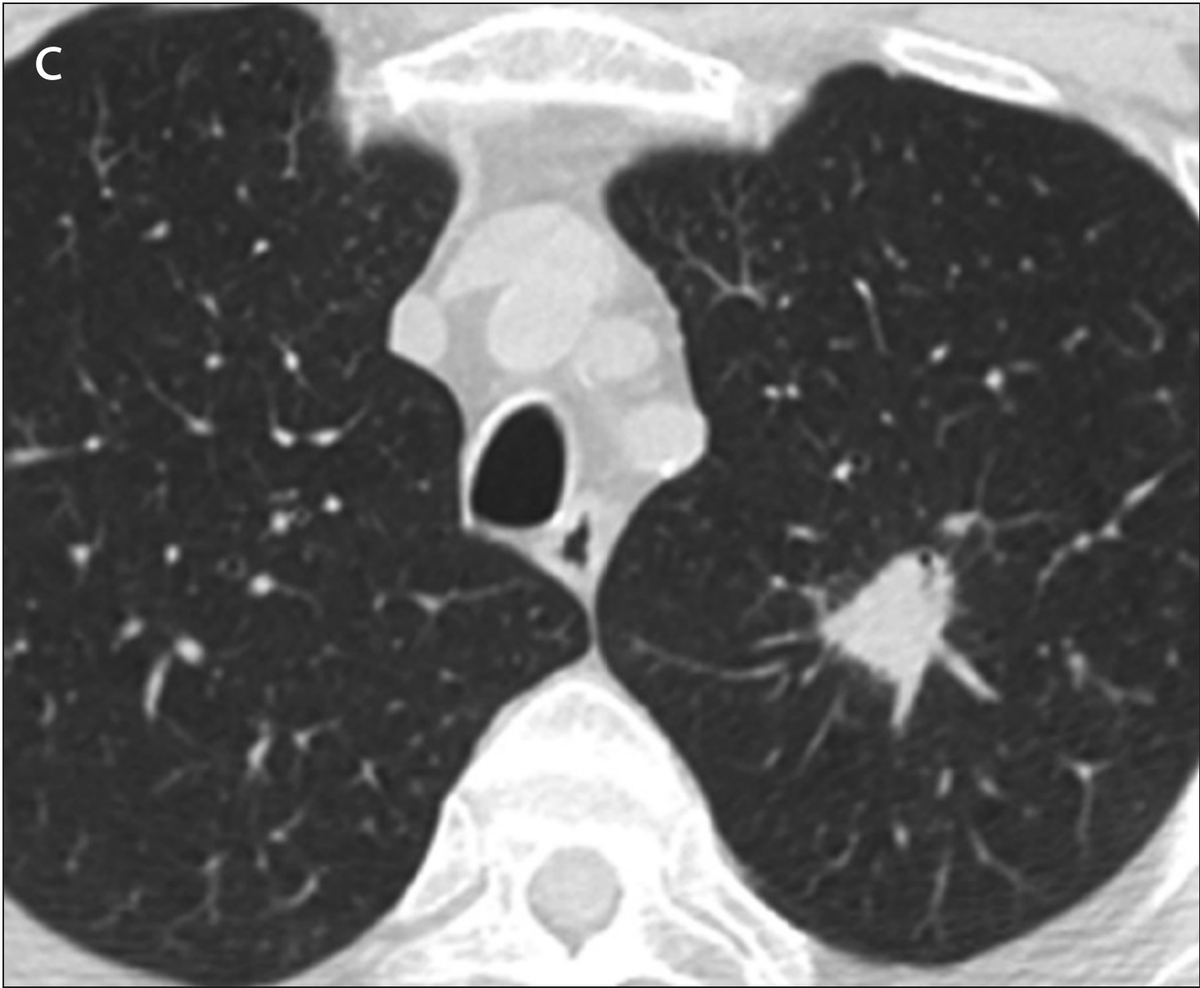
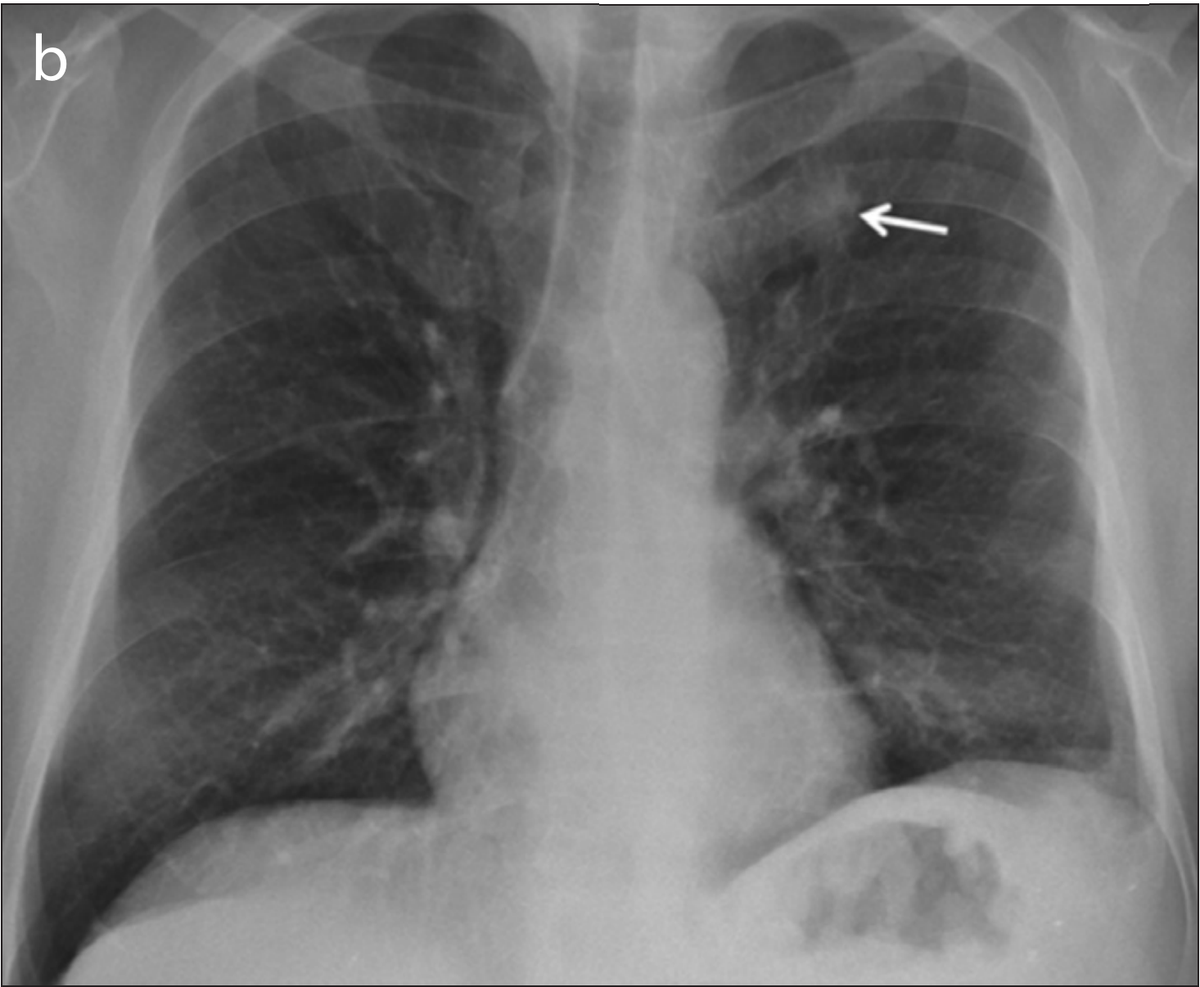
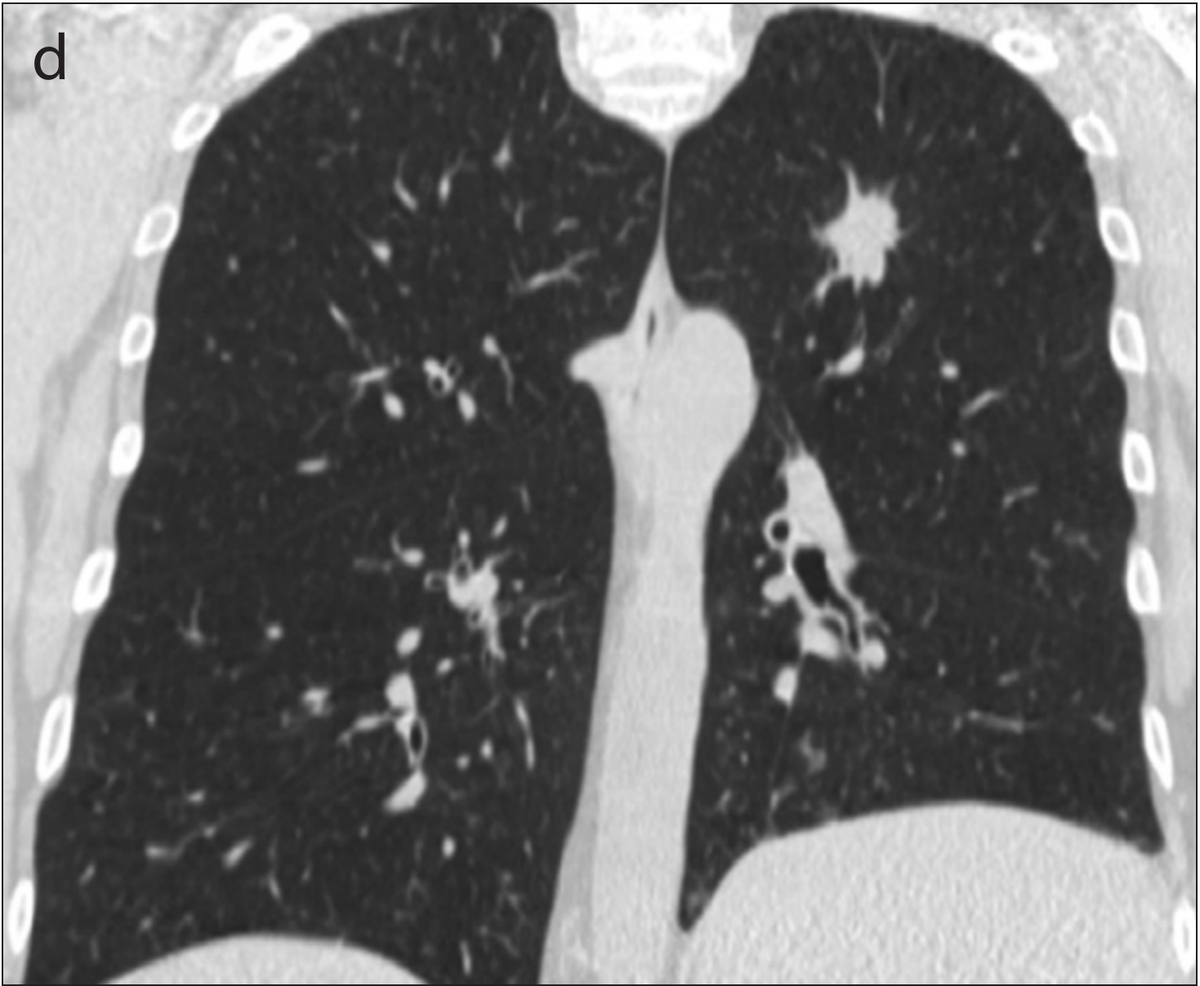
What Can AI Contribute?
The challenge for healthcare providers has been described as "being placed in front of 100 haystacks and told, 'Determine which of these, if any, contains a needle.'" The human mind is simply not well-suited for such work. In contrast, it is precisely the type of task computers excel at.
- The need for early detection has fueled scanning protocols and tools to detect even smaller lung tumors, something of a mixed blessing. Higher resolution scans and more slices also require more effort and attention on the part of radiologists to read them. Allowing AI to review and annotate images shifts some of the burden from busy and overloaded specialists to machines that don't become fatigued or become distracted.
- There are limits to what human vision is able to detect, sometimes causing radiologists to overlook tiny cancerous tumors. AI tools can analyze multiple representations of data (2D slices, 3D volumes, and 3D regions of interest; for example) to help highlight subtle patterns which might not yet be recognized as growth.
- In addition to detecting small nodules, there is also a need for more accurate classifications of suspicious lung nodules as malignant or benign. New AI tools have the ability to determine which features may be of significance and combine features from multiple models to provide estimates of confidence for informing clinical decision-making.
Making Inroads
There is already evidence that computers, helping to augment the abilities of radiologists, may be able to improve the accuracy of early detection. As a starting point, consider that up to 90% of “missed” lung cancer nodules are found when the baseline chest radiograph is re-reviewed providing evidence that technical issues can be overcome and accuracy improved. Computer-assisted diagnosis (CADe) and "AI assistants" (which highlight regions of a scan for further scrutiny) have been shown to improve the accuracy and confidence of a diagnosis.
Recent advances using a type of AI called Deep Learning seem to hold significant promise for providing a "second pair of eyes." Rather than use tumor features defined in advance by a programmer (such as feature textures or Hounsfield unit values), Deep Learning systems figure out for themselves what a tumor is from real examples. A model is given a large dataset of scans (the technique can be used with both CT and x-ray images), some series with nodules and some without. An algorithm then helps the machine learn what a lung cancer tumor looks like, as the model finds the best features to describe a malignant growth.
Multiple research teams have reported success using Deep Learning models to read both x-ray and CT images for early cancer screening. In a 2019 study (Sim et al), researchers from South Korea, Germany, and the United States reported that annotating images with regions of concern on x-rays prior to radiologist review improved the sensitivity of detecting early-stage tumors from 65.1% to 70.3%. It did this while also decreasing the number of false-negative and false-positive findings.
The second image in example 1 (taken from the 2019 Sim paper) highlights how Deep Learning models can help radiologists focus on a region of concern by providing an AI-generated annotation. While three of the twelve radiologist reviewers missed the tumor without the annotation present, all twelve reviewers were able to identify its presence with AI assistance.
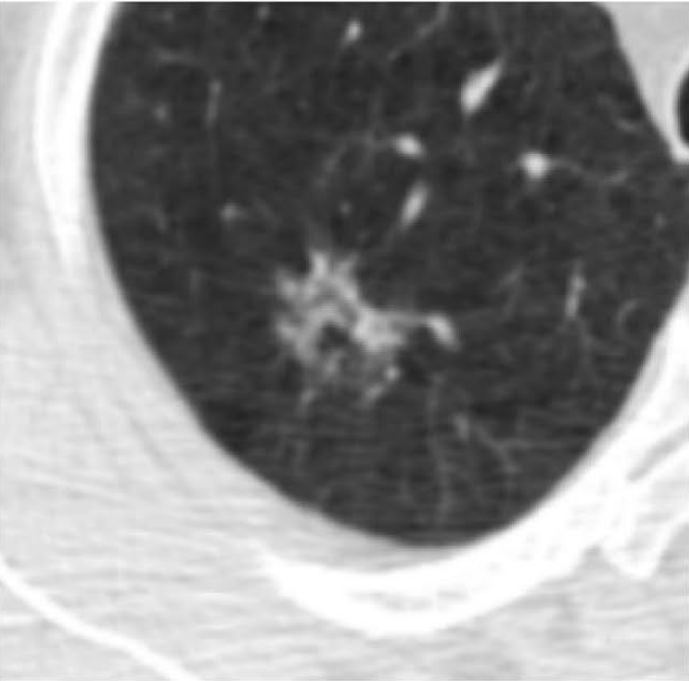
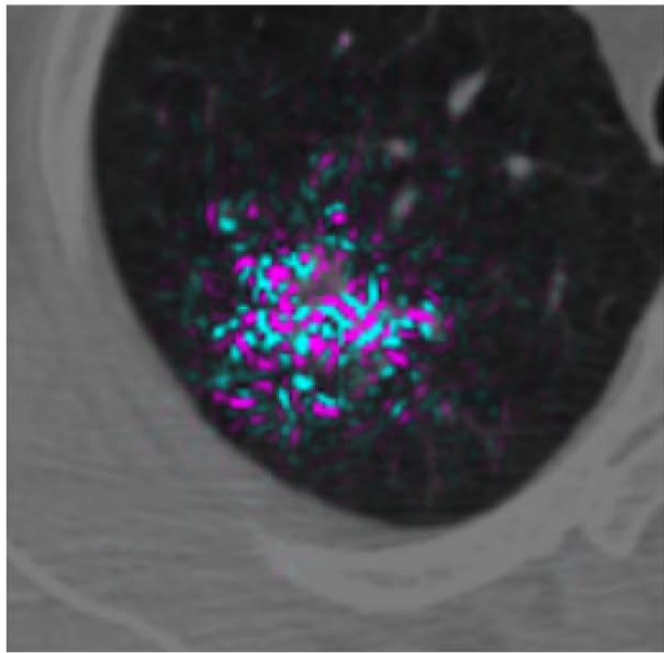
Another 2019 study (Ardila et al), a collaboration between Google's Deep Mind and Northwestern University, reported promising results for better detecting small tumors in CT. The researchers created a three-dimensional AI model that was able to take into account regions of concern within the lung. Making use of both current scans and those from before receiving a lung cancer diagnosis, the model showed good accuracy (94%) in detecting cancerous nodules; outperforming six radiologist reviewers while also reducing the number of false positives and false negatives.
The model's ability to analyze a volume, rather than "merely" a sequence of two-dimensional slices appears to have provided a benefit. The context of the additional pixels allowed the model to inspect patterns in blood vessels and other connective tissue not part of the tumor. This caused the model to highlight contributing factors not directly part of the growth, but capable of providing insight into its development and better predictive accuracy.
So ... What?
Even though lung cancer screening is a very difficult task, with issues caused by the use of x-ray as a first-line tool and human error; there is a great deal of hope that AI can improve the early detection of lung cancer. CADe and AI assistants already show a great deal of promise to call attention to features which may represent small tumors on both x-ray and CT, provide AI generated annotations to ensure that all regions of concern are reviewed, reduce both false-positive and false-negative rates, and help to provide confidence to physicians who may not be thoracic radiologists.
Thus, while still early in their development, it seems possible to answer the first question of this series: AI technologies seem capable of providing a very effective "second pair of eyes." Points to consider when implementing solutions:
- There is no single "correct" modality for cancer screening, and AI models for both x-ray and CT are needed. While CT is better suited for detection, chest x-rays are far more common. For that reason, even a mediocre x-ray model might help locate patients whose cancer might otherwise go undetected and allow confirmation through more sensitive screening methods.
- Models should be integrated into radiology workflows and (where possible) provide additional information such as annotations or suggested findings for radiologists reviewing scans. This approach could help alleviate human errors such as inaccurate interpretation, failure to recognize important findings, and satisfaction of search; and inform clinical decision making.
- When implementing models, it is better to focus on multi-stage workflows and "stacking" techniques where the outputs of one layer might become the inputs of another.This approach also allows for the model to incorporate previous scans or other data into the set of features alongside the imaging series. This is approach appears to be one important reason for the Google Deep Mind model's excellent performance.
- When working with volumetric data, 3D architectures appear to offer benefits over models which work on a 2D series of slices.
In Part 2, we'll turn to a consideration of: "What is the broader context of AI in clinical practice, and what impact might we expect AI solutions to have on treatment?"
Comments
Loading
No results found